Share Share Share Share Email Artificial Intelligence (AI) continues to transform how businesses understand and respond to user behavior. In recent groundbreaking research, Ankit Awasthi highlights significant innovations in adapting transformer architectures specifically for modeling user behavior. These advancements have profound implications for predictive analytics and customer experience optimization.
As a researcher specializing in AI-driven event processing, my exploration into these transformative technologies offers insights into the evolving nature of digital interactions. The Emergence of Adaptive Transformer Models Originally designed to revolutionize natural language processing (NLP), transformer models excel due to their parallel computation capabilities and proficiency in capturing long-range dependencies. Today, these powerful models are being adapted beyond language processing to tackle the complexities of user behavior analysis.
Leveraging self-attention mechanisms, adaptive transformer architectures effectively interpret sequential user interactions, uncovering valuable insights traditional approaches often overlook. Decoding Temporal User Patterns One major innovation in user behavior modeling involves capturing temporal dependencies—something conventional models struggle with. Unlike language, user behaviors occur in dynamic sequences, involving variable time intervals and simultaneous events.
Advanced positional encoding techniques and specialized attention layers empower these AI models to discern both short-term actions and long-term behavioral trends with impressive accuracy. Recent developments introduce multi-resolution temporal modeling, enabling AI systems to simultaneously capture events ranging from microsecond interactions to patterns spanning several months. These models use adaptive time-decay functions, which assign greater importance to recent and relevant events, paired with efficient memory mechanisms to retain essential historical context.
Additionally, integrating causal inference techniques helps distinguish correlation from causation within behavioral sequences, allowing nuanced and actionable predictions about future user behaviors. Hybrid Models: Integrating Temporal Convolution Networks Hybrid architectures integrating temporal convolution networks (TCNs) with transformer-based attention mechanisms have emerged as particularly effective solutions. These models excel at capturing both local temporal patterns and global dependencies, addressing a critical challenge in sequential data analysis.
The combination of TCNs and attention mechanisms facilitates pattern recognition across multiple time scales—effectively identifying immediate user reactions as well as gradual shifts in behavior. This capability is particularly valuable in industries such as retail, where customer purchase decisions evolve over various interactions and touchpoints. Notably, businesses utilizing this hybrid approach report substantial improvements in recommendation systems, customer lifetime value predictions, and churn analysis, resulting in more precise personalized marketing and inventory management.
Beyond Words: An Adaptive Vocabulary for Event Processing While NLP models rely on a fixed vocabulary, behavior modeling requires a dynamic approach. Event-driven AI frameworks redefine vocabulary to capture diverse user actions such as clicks, purchases, and navigation paths. By adopting this adaptive vocabulary, transformer models effectively interpret user intentions and behaviors, refining predictive analytics and enabling richer, more personalized user experiences.
Multi-Dimensional Attention: Creating Comprehensive User Profiles Another notable advancement is the introduction of multi-dimensional attention layers, allowing models to evaluate relationships across multiple data streams simultaneously. Unlike traditional linear attention models, these innovative layers aggregate and analyze data from diverse digital touchpoints, creating richer and more context-aware user profiles. This development significantly enhances personalization and retention strategies by providing a unified view of user interactions across various platforms.
Real-Time Personalization and Experience Optimization AI-driven behavioral analytics is rapidly transforming real-time user experience optimization. Adaptive transformers now process live data streams, dynamically adjusting digital interfaces and content recommendations based on immediate user interactions. This capability is especially impactful in customer service environments, where predictive AI can proactively identify user needs and provide targeted solutions before issues arise.
Looking Ahead: The Future of AI in Behavioral Modeling As transformer-based AI continues evolving, we anticipate further integration of sophisticated techniques such as hierarchical attention mechanisms and reinforcement learning. These advancements promise even greater accuracy and nuance in behavioral predictions. Industries ranging from finance to healthcare stand to benefit significantly from AI’s growing capability to understand and predict complex human behaviors.
In conclusion, Ankit Awasthi ’s research emphasizes the transformative potential of AI-driven event processing. Through specialized transformer architectures, businesses can achieve deeper insights into user behavior, optimize customer journeys, and create highly personalized digital experiences. As AI technology progresses, its pivotal role in shaping strategic user engagement will only expand, ushering in a new era of intelligent decision-making.
Related Items: AI , Ankit Awasthi Share Share Share Share Email Recommended for you Revolutionizing Observability: How AI is Transforming Distributed Systems Using AI, TraceGains Aims To Optimize Food Quality And Safety Creative Intelligence: Where AI and Logo Design Converge Comments.
Technology
Transforming User Behavior Analysis with AI-driven Models
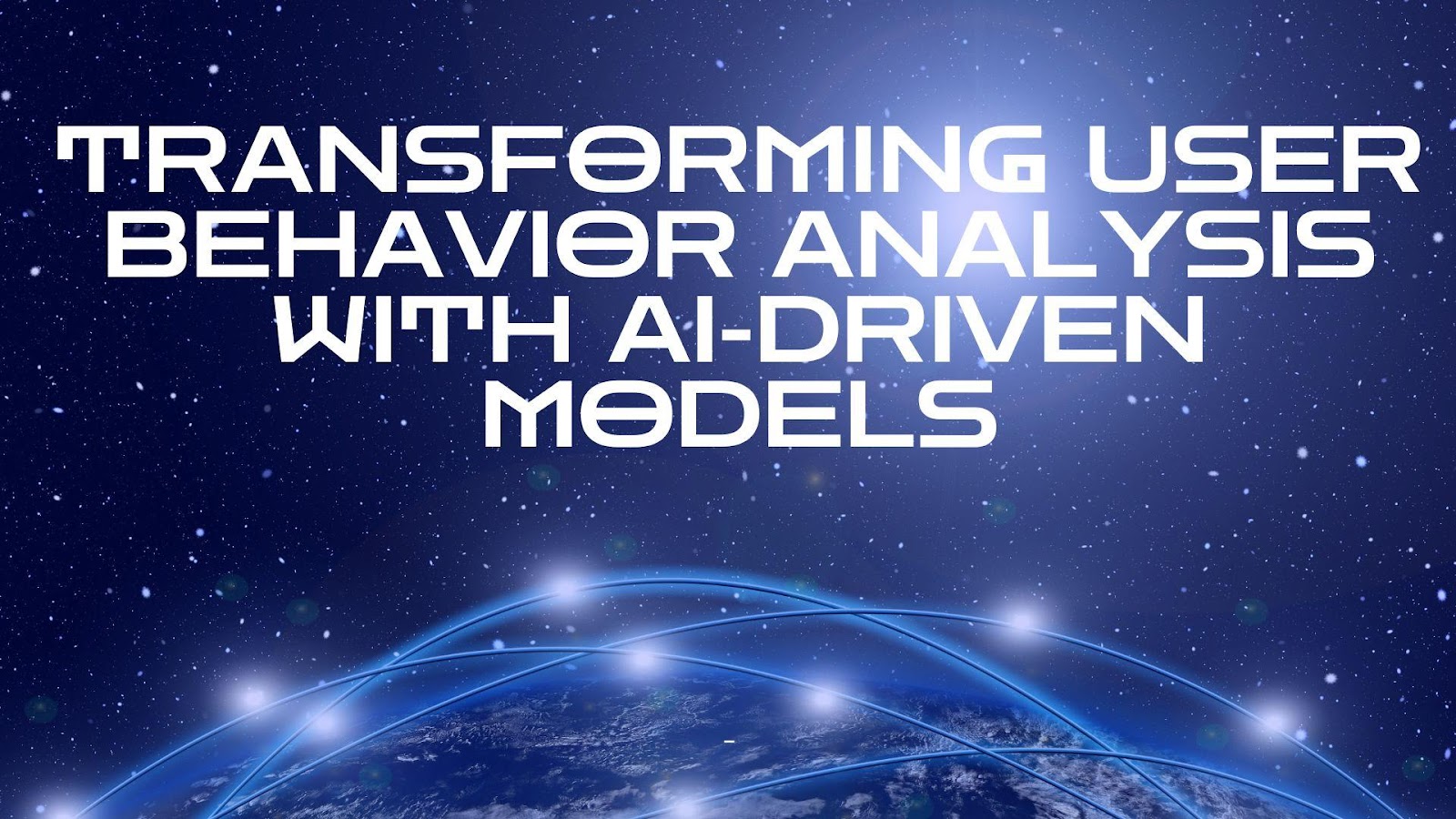
Artificial Intelligence (AI) continues to transform how businesses understand and respond to user behavior. In recent groundbreaking research, Ankit Awasthi highlights significant innovations in adapting transformer architectures specifically for modeling user behavior. These advancements have profound implications for predictive analytics and customer experience optimization. As a researcher specializing in AI-driven event processing, my exploration into [...]The post Transforming User Behavior Analysis with AI-driven Models appeared first on TechBullion.