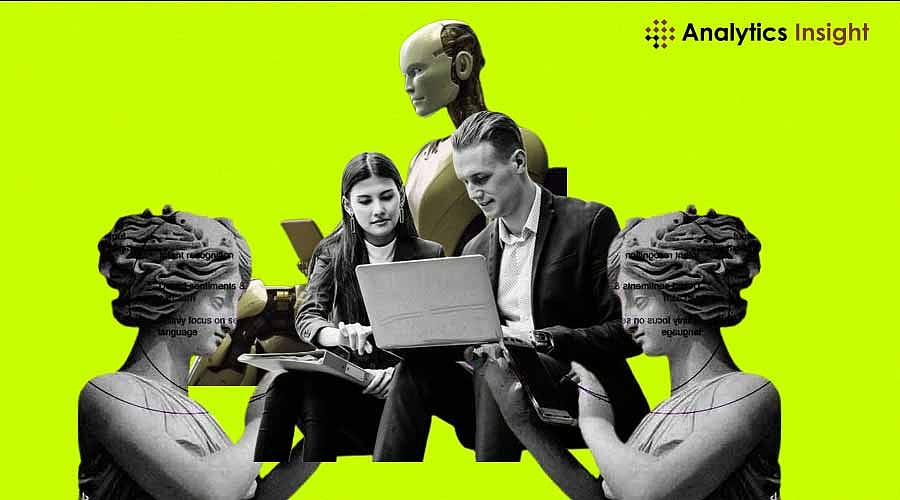
Edge Computing has found its space at the top among transformative technologies for AI workloads. It ensures minimal latency and maximizes real-time decision-making by processing data right near the source, offloading the centralized data center. In this article, we share insight into some of the best edge computing solutions for AI workloads, elaborating on their features, benefits, and application areas.
The NVIDIA Jetson family is the world's leading AI platform for scalable edge AI workloads, including high-performance computing in a small form factor. Jetson is empowering a new generation of diverse applications—from robots to smart cities—all around the globe. The Jetson family includes modules such as Jetson Nano, Jetson TX2, and Jetson Xavier for a variety of performance and power needs.
Key Features: AI Accelerated Server with High-Performance GPU Supports Multi-AI framework: TensorFlow, and PyTorch. Energy-efficient design; hence fitting for embedded systems. Applications: Autonomous robots and drones.
Intelligent video analytics. Industrial automation. The former is an ASIC that is purpose-built to support the execution of AI models at the edge.
TensorFlow is optimized for running well, and, hence, Edge TPU forms very strong hardware to deploy machine learning models at the edge in terms of performance and low power, practically suitable to a wide scope in edge AI applications. Key features: High throughput for AI inference tasks. Low power consumption, perfect for battery-driven devices.
Integration with Google Cloud services in one click. Applications: Home automation devices. Retail analytics.
Healthcare monitoring systems. Intel Movidius Myriad X is a vision processing unit featuring inbuilt support from AI training to inferencing on the edge. It has a dedicated neural computing engine to accelerate loads in deep learning.
Myriad X finds applications in uses that demand real-time processing, especially in image and video processing. Key features: Dedicated neural computing engine for AI workloads acceleration Support for a variety of neural network architectures Low power consumption with a low footprint Applications: Augmented reality devices Autonomous vehicles Surveillance cameras. With AWS Greengrass, the capabilities of AWS can be extended to edge devices.
They are empowered to act locally on the data they create while state-of-the-art cloud power can manage, analyze, and store data relevant to the work done by these devices. What is important is that Greengrass supports machine learning inference to run AI models locally on devices, on the edge. Key Features: Seamless integration with AWS cloud services Local execution of AWS Lambda functions Wired and secure connection between devices and the cloud Applications: Industrial IoT Smart Agriculture Connected Healthcare Azure IoT Edge is a managed service created for data processing and analytics at the edge.
It was designed to support containerized workloads such as AI models which can be deployed and managed from the Azure cloud. In specific detail, it now tightly integrates with other services under Azure, such as Azure Machine Learning. Key Features: Supports containerized AI workloads Integrate easily with Azure Machine Learning and other Azure services.
Strong features when it comes to the security of the edge devices. Applications: Predictive Maintenance Smart Grid Management Real-time Analytics in Retail IBM Edge Application Manager is an AI, Analytics, and IoT workload management solution that is decentralized and autonomous. Help organizations deploy and manage AI models to the edge autonomously in the 1,000s.
Key Features: • Management of edge applications in an independent system. • Adoption of AI, analytics, and IoT workload scenarios. • Scalable: Edge node deployment for 1k number of sensor devices.
Applications: Business enhancement for the supply chain. Smart city development. Telecommunication edge analytics.
It enables the extraction, transformation, and delivery of data from edge devices into applications. A platform that ensures safety and scalability in deploying models for artificial intelligence at the edge. It is also designed for real-time data processing and analytics.
Key features: Secure extraction and transformation of data Integration with Cisco IoT and networking solutions Scalable architecture for large deployments Applications: Industrial automation Smart transportation systems Real-time monitoring of the energy sectors HPE has innovated the HPE Edgeline by bringing enterprise-class computing to where the data is created with no compromise performance or reliability on AI and machine learning workloads. Key Features: Enterprise-class computing capabilities. AI/ML workload capacity Delivers AI and machine learning workloads No compromise performance and reliability Applications Real-time health care data processing.
Solution for smart retail. The Dell EMC PowerEdge XE2420 is a server powerhouse streamlined for edge computing. It empowers AI and machine learning workloads—with the computational power needed to face up against real-time data processing from the edge.
Key features High computational performance for supporting AI workloads Compact design ideal for edge environments High security and robust management features Applications Edge analytics for retail Industrial setups for real-time monitoring Telecommunications with AI-driven insights Nokia Edge Automation provides an end-to-end solution for managing the AI workloads taken to the Edge. This provides the AI applications with deployment, management, and scalability to ensure their execution effectively and reliably. Key features This includes the tools to manage the entire AI workload.
Scale to large EDGE deployments. Integrated with Nokia network solutions Applications: Smart City Infrastructure Transportation Real-time analytics Embedding AI in Commercial Edge Computing Edge computing solutions lie at the core of executing AI workloads effectively and efficiently at the periphery, hence reducing latency, making advanced real-time decisions, and reducing the load on the centralized data center as data is acted upon at the source..