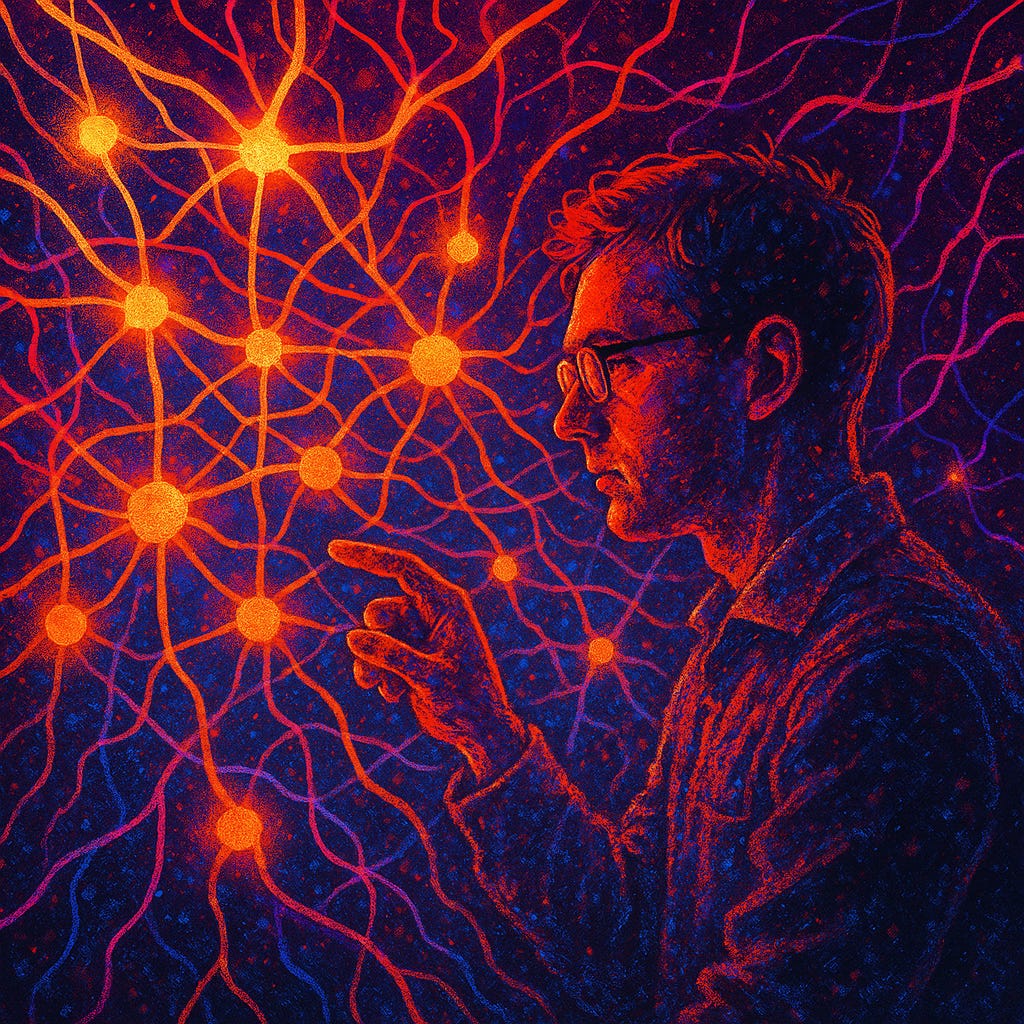
Created Using GPT-4oNext Week in The Sequence:We start a new series about evaluations, cannot miss this one. Our opinion section will debate while MCP is getting so much adoption in the AI space. The research edition will dive into the Anthropic’s new interpretability research.
The engineering section will dive into another cool framework. You can subscribe to The Sequence below:TheSequence is a reader-supported publication. To receive new posts and support my work, consider becoming a free or paid subscriber.
📝 Editorial: The Amazing GPT-40 Image GenerationThis week AI headlines were dominated by the launch of GPT-4o image generation. However, I wanted to dedicate the editorial to two research papers published by Anthropic that could mark a new milestone in AI interpretability. In two papers published last week, Anthropic seems to have made a substantial leap in the field of interpretability in large language models, specifically Claude 3.
5 Haiku. By applying neuroscience-inspired methods, researchers mapped computational circuits within the model—unveiling how Claude processes inputs, reasons through information, and generates text. These insights challenge traditional beliefs about LLMs as mere pattern-matchers and mark a major step toward decoding the black-box nature of modern AI.
One of the most striking findings is Claude’s ability to reason abstractly across languages. When prompted with the concept of opposites—like "small" and its antonym—in various languages, Claude activated abstract features detached from specific linguistic tokens. This implies that the model constructs a shared internal semantic space where concepts exist before being rendered in any particular language.
Interestingly, these language-agnostic modules scale with model size, suggesting larger LLMs are developing more generalized reasoning structures.The research also upends the assumption that LLMs merely generate one word at a time without foresight. Claude demonstrated evidence of forward planning, such as pre-selecting rhyme words (e.
g., “rabbit”) early in poetic composition. In tasks like identifying the capital of Texas, the model activated context-relevant concepts (“Texas”) before stating the answer (“Austin”).
However, not all reasoning chains were authentic—researchers observed instances where the model retroactively generated justifications for pre-decided outputs. This duality reflects both the potential and the pitfalls of LLM-based reasoning.Through dictionary learning, Anthropic uncovered roughly 10 million interpretable features within Claude, each corresponding to recognizable concepts or abstractions.
These features form computational circuits—dynamic pathways that determine how inputs evolve into outputs. A notable discovery was the existence of a “default suppression” circuit, which prevents the model from hallucinating until overridden by certain entity-recognition triggers. While impressive, these 10 million features represent only a fraction of the total, underscoring how much of the model remains uncharted.
With circuit-level transparency comes the potential for more controllable and safer AI. Anthropic’s work allows researchers to pinpoint circuits linked to unreliable or deceptive reasoning. In one case, an internal feature likened to a “racism bot” exhibited suicidal conflict when its assumptions were challenged—revealing both the power and ethical complexity of such insights.
While transformative, these interpretability methods currently require enormous resources, making them impractical for complete analysis of frontier models.Despite the breakthroughs, the path ahead is steep. Today’s tools can only scratch the surface of LLM cognition.
Mapping features doesn’t fully explain their interactions, much like knowing neuron types doesn’t equate to understanding the brain. Still, Anthropic’s work opens doors to real-time auditing, model alignment, and verifiable reasoning in high-stakes applications. As interpretability becomes a frontier of its own, future efforts must focus on scaling these tools to match the models they aim to decode.
By revealing the mechanisms behind Claude’s reasoning, Anthropic transforms AI interpretability from philosophical speculation into actionable science. It’s a pivotal moment for the field—one that could shape not only how we build future LLMs but also how we ensure they remain aligned with human values in the process.🔎 AI ResearchAnthropic InterpretabilityAnthropic published two landmark papers in AI interpretability.
The first paper focuses on locating interpretable concepts and combining them using a concept called computational circuits. This idea reveals specific behaviors about how LLMs arrive to a specific output. The second paper applies those ideas to Claude Haiku to understand some of its specific behavior.
4o Image GenerationIn the system card for "Native Image Generation" (referring to 4o image generation), researchers from OpenAI detail the safety measures and capabilities of their new image generation model34. The system card reports that 4o image generation exhibits less bias than DALL·E 3 across various metrics, although challenges in demographic representation remain, with plans for continued refinement and more diverse training data.Gemini RoboticsIn the paper Gemini Robotics: Bringing AI into the Physical World researchers from Gemini Robotics Team, Google DeepMind introduce a new family of AI models for robotics built upon Gemini 2.
0, including the Vision-Language-Action model Gemini Robotics and the Embodied Reasoning model Gemini Robotics-ER1 . This report details how these models enable robots to understand and interact with the physical world through enhanced spatial and temporal reasoning, allowing for tasks ranging from basic manipulation to dexterous skills, with capabilities like zero-shot and few-shot learning, and adaptation to new robot embodiments.Qwen 2.
5In the paper Qwen2.5-Omni Technical Report researchers from the Qwen Team present Qwen2.5-Omni, an end-to-end multimodal model capable of processing text, images, audio, and video and generating text and natural speech responses simultaneously in a streaming manner3 .
The report introduces the Time-aligned Multimodal RoPE (TMRoPE) for synchronizing multimodal inputs and the Thinker-Talker architecture for concurrent text and speech generation, demonstrating state-of-the-art performance on various multimodal benchmarks and highlighting its speech instruction following and generation capabilities.CaMeL In the paper "Defeating Prompt Injections by Design" researchers from Google DeepMind and ETH Zurich present CaMeL, a novel defense mechanism against prompt injection attacks in language model agents. CaMeL draws inspiration from software security principles, focusing on securing both data and control flows by extracting the intended control flow as pseudo-Python code and executing it with a custom interpreter.
MDocAgentIn the paper "MDocAgent: A Multi-Modal Multi-Agent Framework for Document Understanding" researchers from UNC-Chapel Hill and Adobe Research present MDocAgent, a novel Retrieval Augmented Generation (RAG) and multi-agent framework for Document Question Answering . The key contribution is the integration of five specialized agents that collaboratively leverage both textual and visual information from documents. MDocAgent addresses the limitations of existing methods that often prioritize a single modality or struggle with complex multi-modal reasoning and long documents.
đź“¶AI Eval of the Weeek(Courtesy of LayerLens )LayerLens ran some evaluations against the new DeepSeek v3 0324 with very impressive results. See below the chart for some of the top benchmarks as well as the relevant explanations. The model clearly excels in math and reasoning.
🤖 AI Tech ReleasesMCP in AzureMicrosoft unveiled support for model context protocol(MCP) across different Azure services. DeepSeek-V3-0324DeepSeek released a new version of DeepSeek v3 with major performance improvements. 🛠AI in ProductionScaling AgentforceSalesforce shares some of the best practices for scaling its Agentforce platform across thousands of customers.
Embeddings at AirbnbAirbnb discusses their embedding architecture to power its search capabilities. 📡AI RadarxAI acquired X in a deal that valued xAI at $80 billion and X at $33 billion.SoftBank is finalizing a $40 billion investment in OpenAI.
NVIDIA is in talks to acquire GPU reseller Lepton AI. Amazon Alexa Fund is expanding its investment scope to include AI startups. AI education tech Brisk raised $15 million to integrate AI into classrom experiences.
Hakimo raised $10.5 million to use AI in physical security environments. Paid raised $10 million for building a payment platform for AI agents.
n8n raised $60 million for its AI workflow automation platform. AI chip maker FuriosaAI rejected $800 million from NVIDIA. Browser Use raised $17 million for AI agents that can navigate the web.
.