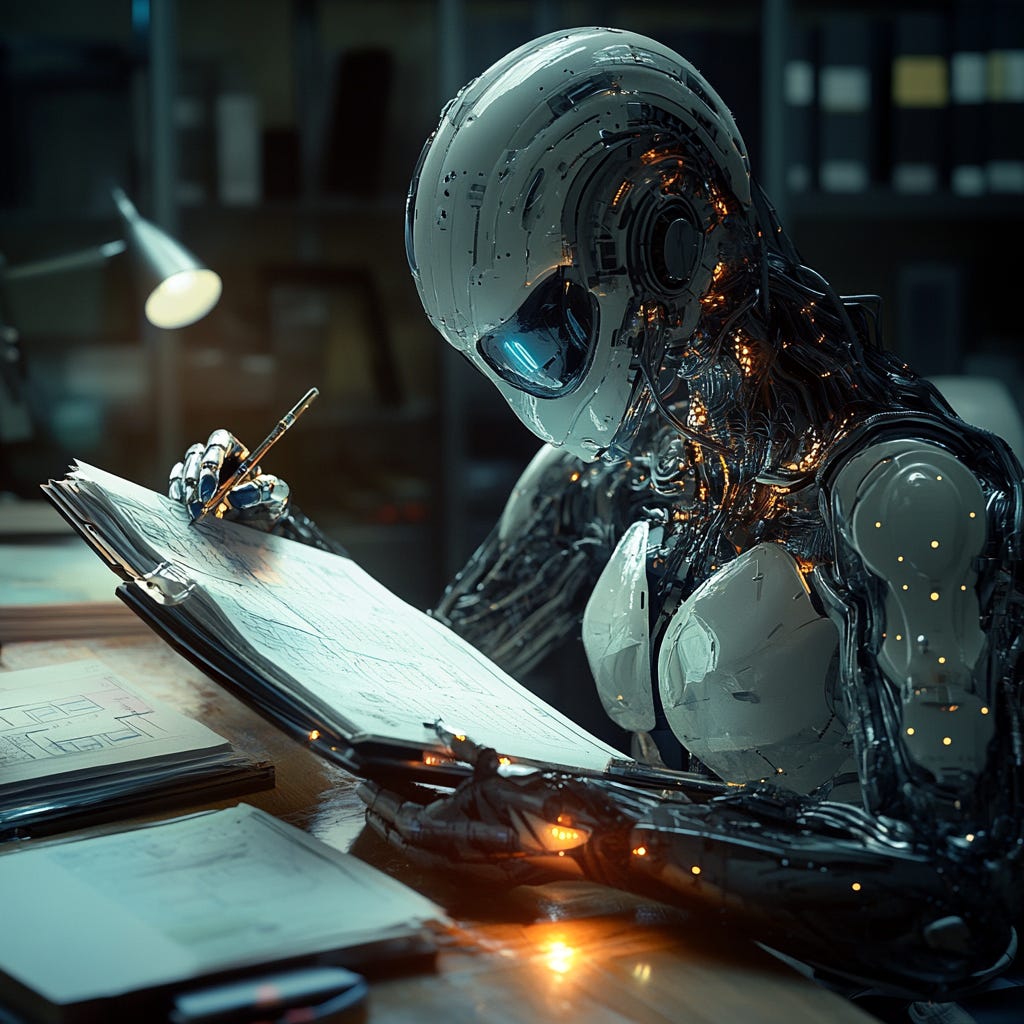
A key differentiator of HLE is its rigorous question development and review process. Each question undergoes multiple stages of scrutiny, including an initial check against state-of-the-art LLMs, and is rejected if LLMs can answer it correctly. Following this initial check, the questions proceed to a two-stage human review.
The first review round involves multiple graduate-level reviewers who iteratively refine the questions. The second round is conducted by organizers and expert reviewers who approve questions based on quality and adherence to submission criteria. This multi-stage review process ensures that only the most challenging and high-quality questions are included in the benchmark.
Additionally, all questions must have a known solution that is unambiguous and easily verifiable. This meticulous approach to question creation is a key contribution, as it helps ensure that the benchmark measures advanced reasoning and knowledge, rather than susceptibility to memorization or retrieval. Another key contribution of the HLE benchmark lies in its diverse question formats and subject coverage.
The benchmark includes both exact-match and multiple-choice questions, as well as multi-modal questions that require comprehending both text and image references. This variety of formats ensures that models are evaluated across a broader range of skills. Furthermore, HLE spans a wide array of academic subjects, from STEM fields to law, history, and the arts.
This breadth of subject matter ensures that the benchmark is a holistic measure of overall academic ability. By incorporating this wide variety of questions, HLE moves beyond subject-specific tests, aiming to provide a more complete assessment of an LLM's knowledge and problem solving capabilities. The evaluation results of HLE demonstrate its efficacy as a challenging benchmark.
State-of-the-art LLMs consistently show low accuracy (less than 10%) and poor calibration on HLE, indicating a substantial gap between current model capabilities and expert-level performance. Models often provide incorrect answers with high confidence rather than acknowledging their uncertainty, which highlights the problem of hallucination. This level of difficulty contrasts with the saturation seen in many existing benchmarks, demonstrating the utility of HLE in assessing frontier AI capabilities.
Furthermore, the evaluation setup includes a standardized system prompt that structures model responses, as well as GPT-4O as a judge to verify answer correctness, ensuring consistency and objectivity..