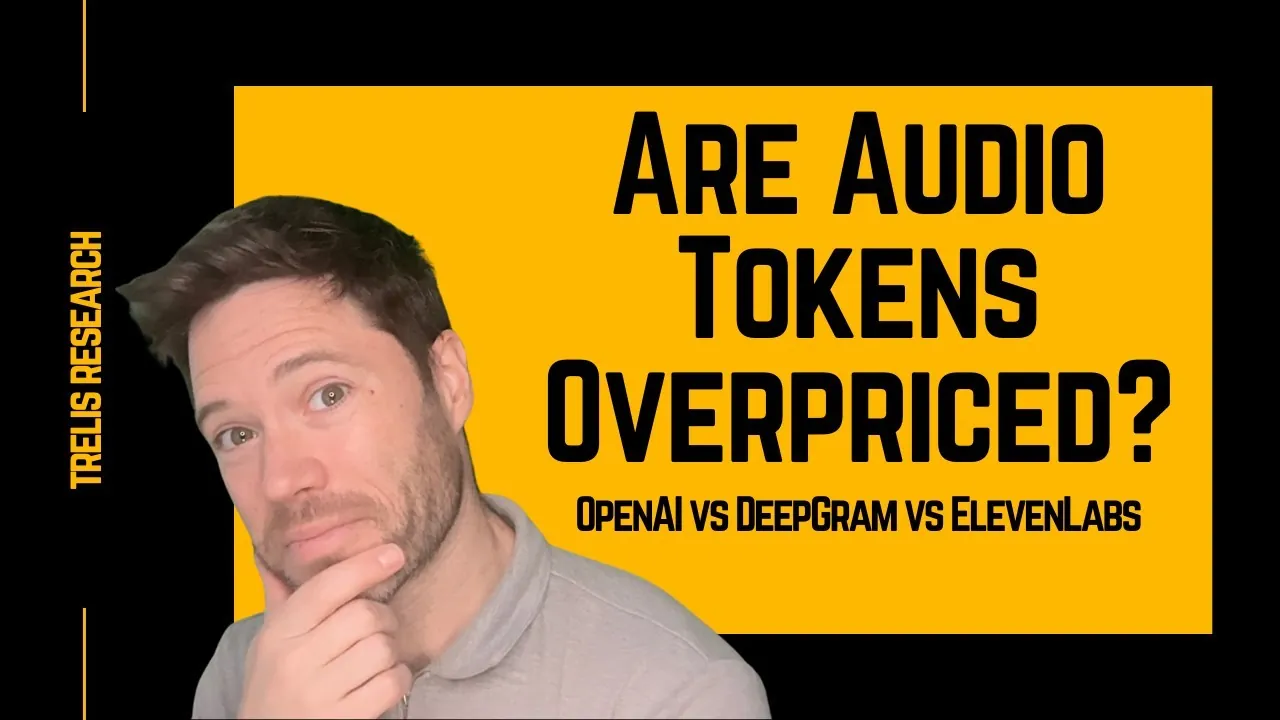
The audio processing industry is witnessing a dynamic shift as leading players like OpenAI, ElevenLabs, and DeepGram compete to establish dominance. This competition is driving a concerted effort to reduce costs for text-to-speech (TTS), speech-to-text (STT), and conversational AI services. These advancements are not only reshaping the audio token costs and economics of audio processing but also paving the way for broader adoption of these technologies across industries.
By examining pricing strategies, technological innovations, and market trends, Trelis Research helps you better understand the forces shaping the future of audio tokenization. Audio tokenization is computationally expensive due to the high density of tokens required for processing audio data compared to text, driving up operational costs for TTS, STT, and conversational AI services. OpenAI, ElevenLabs, and DeepGram have distinct pricing strategies: OpenAI Whisper offers premium STT services, ElevenLabs focuses on high-quality TTS at a higher cost, and DeepGram provides more affordable STT solutions.
Open source models like Fireworks and MOSI are disrupting the market by offering comparable performance at lower costs, creating downward pressure on proprietary pricing structures. Multimodal models, such as OpenAI’s GPT-4, are emerging as a potential solution to integrate audio, text, and visual data, but achieving real-time performance at lower costs remains a challenge. While TTS and STT costs are expected to decline due to advancements in efficient models, conversational AI will likely remain a premium service due to the complexity of real-time reasoning and natural language understanding requirements.
Audio tokenization involves converting audio data into machine-readable tokens, allowing AI models to process and analyze sound. This process is far more resource-intensive than text processing due to the sheer volume of data involved. While a single sentence in text may require only a handful of tokens, processing one second of high-quality audio can demand hundreds of tokens.
This disparity underscores the higher computational requirements and operational costs associated with audio models. High-quality audio models, such as OpenAI’s Whisper, rely on advanced token generation techniques and significant computational power. These requirements contribute to the elevated costs of audio services, particularly in real-time applications like conversational AI.
However, recent advancements in smaller, more efficient models are beginning to challenge these cost structures. These innovations offer the potential for more affordable solutions without compromising performance, signaling a shift in the industry’s approach to cost management. The pricing strategies of OpenAI, ElevenLabs, and DeepGram reflect their unique market positions and priorities.
Each provider has tailored its offerings to balance performance, quality, and cost, catering to different user needs. OpenAI’s Whisper is known for its robust STT capabilities, offering competitive rates for premium services. While its pricing is higher than some open source alternatives, it reflects the proprietary nature and high performance of its models.
ElevenLabs stands out for its natural-sounding TTS solutions, which prioritize audio quality. However, this focus on quality comes at a premium, making it the most expensive option among the three providers. DeepGram appeals to cost-conscious businesses with its affordable STT solutions.
While its pricing is competitive, the company may need to further adjust its rates as the market continues to evolve. In addition to these providers, open source models like Fireworks and MOSI are gaining traction. These alternatives offer comparable performance at a fraction of the cost, exerting downward pressure on pricing across the industry.
As open source solutions become more sophisticated, they are likely to play an increasingly significant role in shaping the competitive landscape. Dive deeper into AI voice and audio with other articles and guides we have written below. Several key trends are driving the push to lower audio tokenization costs.
One of the most significant is the rise of open source audio models. Models such as Orus and CSM 1B demonstrate that smaller, more efficient architectures can deliver high-quality results without the steep computational demands of larger models. This shift toward efficiency is expected to accelerate as developers prioritize scalability and cost-effectiveness.
Another important development is the emergence of multimodal models that integrate audio, text, and visual data. OpenAI’s GPT-4, for example, highlights the potential of unified models to streamline processing and reduce costs. However, these models face challenges in achieving real-time reasoning while maintaining affordability.
As the industry continues to innovate, balancing these competing demands will be critical to the success of multimodal solutions. The pricing strategies employed by TTS and STT providers reveal significant profit margins, particularly for premium services. For example, ElevenLabs charges a premium for its high-quality TTS offerings, while OpenAI’s audio services are priced higher than their text-only counterparts.
These pricing discrepancies highlight opportunities for optimization and cost reduction, particularly as competition intensifies. For businesses, especially startups and smaller enterprises, the high costs of audio services can pose a barrier to adoption. However, as more efficient models become available and providers adjust their pricing strategies, these technologies are likely to become more accessible.
This shift could enable a wider range of industries to use audio processing capabilities, driving innovation and growth across sectors. Conversational AI represents one of the most complex and resource-intensive applications of audio processing. These systems require large, sophisticated models capable of real-time reasoning and natural language understanding.
As a result, the costs associated with conversational AI are unlikely to decrease as rapidly as those for TTS and STT services. OpenAI’s multimodal models, which integrate audio with other data types, may offer a competitive advantage in this space. By balancing advanced reasoning capabilities with real-time performance, these models could help address some of the cost challenges associated with conversational AI.
However, achieving significant cost reductions will require continued innovation in model efficiency and computational optimization. The future of audio token cost is poised for significant transformation. As smaller, more efficient models gain traction, the costs of TTS and STT services are expected to decline substantially.
Open source initiatives will play a pivotal role in this shift, providing affordable alternatives to proprietary models and fostering greater competition in the market. Conversational AI, however, is likely to remain a premium service due to the complexity of the models involved. Providers will need to innovate continuously to balance performance with affordability, making sure these technologies remain accessible to a diverse range of users.
As the industry evolves, the interplay between proprietary and open source solutions will shape the trajectory of audio processing costs, offering new opportunities for businesses and developers alike. Media Credit:.