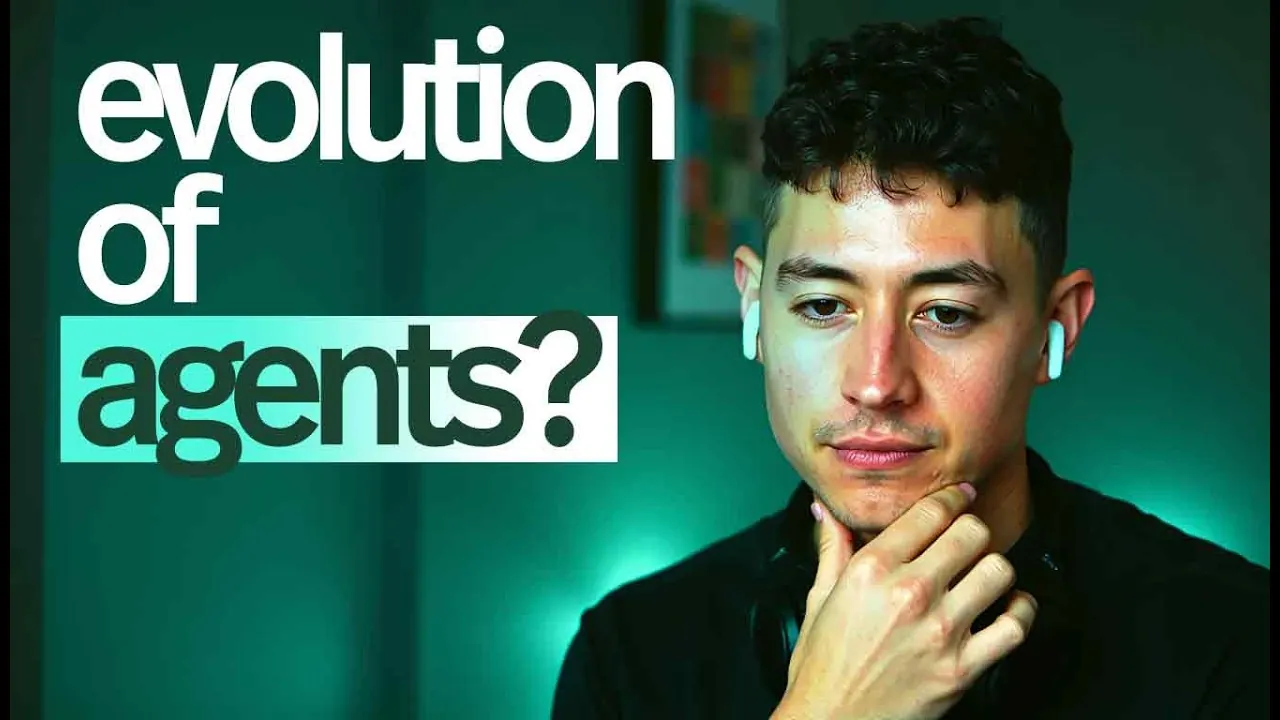
The Model Context Protocol (MCP) is poised to redefine how artificial intelligence (AI) agents interact with external tools and services. Imagine a world where connecting AI systems to and services is as simple as flipping a switch. No more wrestling with tedious configurations, troubleshooting errors, or worrying about scalability as your automation needs grow.
If you’ve ever felt the frustration of piecing together complex workflows or wished for a more seamless way to integrate AI into your processes, you’re not alone. with the tools we rely on every day. But what exactly is MCP, and how does it work? While it’s still in its early stages, MCP has already captured the attention of major players like Anthropic and OpenAI for its potential to transform AI-driven automation.
By automating the configuration of tool connections and making sure secure, , MCP promises to eliminate many of the headaches that come with traditional integration methods. Whether you’re an automation enthusiast or just dipping your toes into the world of AI, this article will break down MCP in plain terms—no hype, no fluff—so you can understand what it means for the future of automation and how it might fit into your workflows. MCP (Model Context Protocol) standardizes AI-agent interactions with external tools, simplifying workflows, reducing manual configurations, and enhancing scalability and accuracy in automation.
Its core components include MCP Clients, MCP Servers, and External Services, allowing secure and streamlined communication between AI systems and tools like CRMs, databases, and email platforms. MCP offers key benefits such as streamlined configuration, error reduction, simplified workflows, and scalability, making it a valuable tool for automation professionals. Despite its potential, MCP is in its early stages, with limited server availability, technical setup challenges, and an immature ecosystem, making it unsuitable for production-level use currently.
The future of MCP is promising, with anticipated growth in server development, platform integration, and potential standardization, offering significant opportunities for automation professionals to gain a competitive edge. MCP is a protocol designed to standardize and streamline the interaction between AI agents and external tools. It automates the configuration of tool connections, enhances security, and ensures consistent input schemas.
This eliminates the need for manual setup, making it easier for AI systems to interact with databases, customer relationship management (CRM) platforms, email systems, and other external services. Originally developed by Anthropic, MCP has gained traction with support from OpenAI, signaling its growing importance in the AI ecosystem. By automating traditionally manual processes, MCP reduces the complexity of integrating AI agents with external tools.
For example, instead of manually configuring connections for each tool, MCP provides a unified framework that ensures secure and standardized communication. This approach not only saves time but also reduces the likelihood of errors, making it an essential tool for automation professionals. MCP functions through a structured framework that assists seamless communication between AI agents and external services.
Its core components include: Serves as the interface between the AI agent and MCP servers, processing requests and making sure secure communication. Manages tool calls, formats API requests, and interacts with external services. Tools such as databases, CRMs, and email systems that are accessed through MCP servers.
The process begins with MCP servers providing a list of available tools to the MCP client at runtime. The AI agent selects the appropriate tool from this list, while the MCP client ensures secure and standardized communication. This structured approach reduces errors, improves efficiency, and simplifies the integration process, making it easier to scale AI-driven workflows.
Here are more detailed guides and articles that you may find helpful on Model Context Protocol (MCP). MCP offers several key benefits that make it a valuable tool for AI-driven automation: Eliminates the need for manual setup of individual tool nodes, saving time and reducing complexity. Standardized prompts and schemas minimize the risk of miscommunication between AI agents and external tools.
Particularly advantageous for complex automation tasks that involve multiple tools and services. Enables AI agents to access multiple tools through a single, unified connection. These benefits make MCP an attractive option for automation professionals seeking to optimize their processes, improve system reliability, and reduce the time spent on manual configurations.
Despite its potential, MCP is still in the early stages of adoption. Experimental implementations are available in platforms like n8n and Cursor AI, but robust integrations with major tools such as Salesforce and Google Workspace remain limited. Additionally, many MCP servers are still under development, with restricted functionality and occasional bugs.
These limitations make MCP unsuitable for critical production-level projects at this time. Several challenges must be addressed for MCP to achieve widespread adoption: High-quality MCP servers for popular tools are scarce, limiting its practical applications. Platforms like n8n require allowing community nodes, which can be a barrier for less technical users.
The protocol is still evolving, and its ecosystem lacks the maturity needed for large-scale deployment. Addressing these challenges will require investment in server development, broader support from AI and automation platforms, and continued refinement of the protocol. The future of MCP is promising, with several developments on the horizon that could significantly enhance its adoption and utility: Growth in MCP servers for major SaaS platforms like Salesforce, HubSpot, and Google Workspace is expected.
Potential adoption by AI development platforms such as Zapier, Make.com, and Lindy could accelerate its use. MCP has the potential to become the default protocol for AI-agent-to-service communication, streamlining automation across industries.
As the ecosystem matures, MCP could transform how automation professionals design and implement AI-driven workflows, offering a more efficient and scalable alternative to traditional methods. For automation professionals, MCP presents unique opportunities to gain a competitive edge in the evolving landscape of AI-driven automation: Use MCP internally to gain hands-on experience and explore its potential applications. Develop and monetize MCP servers for popular tools, positioning yourself as an early adopter and innovator.
Use MCP-based solutions to stand out in the competitive automation market. By understanding MCP’s capabilities and preparing for its broader adoption, automation professionals can position themselves as leaders in the field, ready to capitalize on the protocol’s growing significance. MCP simplifies AI automation by reducing the complexity of connecting tools and services.
It offers a more efficient alternative to traditional node-based configurations in platforms like n8n, allowing faster and more reliable automation workflows. While its long-term benefits are clear, the ecosystem is still maturing. For now, automation professionals should focus on building familiarity with MCP, experimenting with its capabilities, and staying informed about its ongoing development.
As MCP continues to evolve, its adoption by major players like OpenAI and Anthropic underscores its potential to become a foundational standard in AI automation. By staying ahead of the curve and embracing MCP’s possibilities, automation professionals can unlock new opportunities and drive innovation in their workflows. Media Credit:.