New Delhi: India’s dream of Atmanirbharta in defence has long been slowed down by its inability to master aircraft engine technology. A new sector where the country may not be able to compete fiercely is the artificial intelligence space. The AI race cannot be won without high-performance chips, and India remains dependent on foreign graphics processing units (GPUs), indicating strategic and technological vulnerability.
Sunil Gupta, co-founder, MD and CEO of Yotta Data Services—empanelled under IndiaAI Mission to provide compute facilities—told ThePrint that “India has a strong foundation in software and AI talent, but scaling AI models to a global level requires advanced computational power, semiconductor access, and sustained investments in high-end GPUs”. As companies sourcing GPUs under the government’s AI Compute Portal initiative are set to receive their AI workloads—tasks performed by AI systems, including data processing, model training and other AI-based applications—this month, ThePrint mapped the GPU chokepoints in India’s AI landscape, exploring how the country is navigating infrastructure challenges across industries and academia. Launched by Union IT Minister Ashwini Vaishnaw on 6 March, 2025, as part of the IndiaAI Mission, the AI Compute Portal aims to enhance accessibility and affordability of GPUs for AI researchers, academic institutions and businesses.
GPUs, which were initially designed for gaming purposes, have now evolved into indispensable components of AI. Their ability to break down complex tasks into smaller simultaneous computations makes them vital for training AI models, running simulations and handling vast datasets. But as nations race to secure their own chip supply, GPUs have transformed from mere technology to a strategic asset.
Countries like the US, China and Taiwan, with their control over advanced semiconductor manufacturing, hold a significant geopolitical advantage. For India, heavy reliance on imported GPUs poses risks associated with national security, data privacy, supply chain disruptions and sanctions, which in future could stall the country’s AI ambitions. “India must develop sovereign AI infrastructure, expand its data centre capacity, and foster deeper public-private partnerships to ensure self-reliance, as strategic investments in AI compute infrastructure and indigenous semiconductor capabilities will be critical in positioning India as a global AI hub,” Gupta said.
According to government data, GPUs worth over $29 million were imported over the last five years from the US, China and Taiwan. Infographic: Manali Ghosh | ThePrint The global GPU landscape is dominated by a handful of players. While American companies, like NVIDIA and AMD (Advanced Micro Devices) lead in design, Taiwan’s TSMC (Taiwan Semiconductor Manufacturing Company) and South Korea’s Samsung are top manufacturers.
The government’s new initiative, IndiaAI Compute Portal, will offer AI compute, network, storage, platform and cloud services at discounted rates to startups, MSMEs, researchers and government agencies. “Indian computing ecosystem is poised for a significant and transformative shift. By lowering the cost barrier, this approach accelerates AI R&D efforts and plays a crucial role in stimulating local innovation,” Ranjit Metrani, president of managed services at CtrlS Datacenters, one of the first few companies to be assigned AI workload, told ThePrint.
These government-procured GPUs will be available at a highly subsidised rate of less than Rs 100 per hour, significantly cheaper than the global average of $2.5-3 per hour. Additionally, there are plans to develop GPUs indigenously within the next three to five years.
Also Read: No more ‘babying’ robots. Columbia University researchers teach them to watch & learn India’s lack of indigenous capabilities in GPU production has led to overwhelming import dependency. Data service providers, like Yotta and Neysa.
AI, therefore import GPUs, and make them accessible to startups and research institutions for training models, running data centres and building AI-based services. “At Yotta, GPUs are at the core of our AI and High-Performance Computing offerings. With Shakti Cloud, powered by NVIDIA H100 GPUs, we provide businesses and researchers with scalable AI computing resources.
Our GPU-as-a-Service model ensures cost-effective, on-demand access to high-performance compute power,” Yotta CEO Gupta said. GPUs are high-cost resources, especially those designed for data centre applications, which handle large amounts of data to train AI models and run AI-based applications. In India, the price of data centre-grade GPUs can start at around Rs 4.
3 lakh, and go up to Rs 1.46 crore or more, depending on performance and specification requirements. NVIDIA follows a rigorous selection process, assessing buyers on factors like intended applications, data center readiness and scalability.
“NVIDIA evaluated our capabilities before approaching us a few years ago. Our advanced data center capacity, power infrastructure, fiber connectivity, and expertise in managing GPU services played a key role in securing access to Nvidia GPUs,” Gupta told ThePrint. The US-based chipmaker dominates this space of GPU research and design.
Its dominance stems not just from its hardware design capabilities, but also from its software platform—CUDA (Compute Unified Device Architecture). NVIDIA’s CUDA programming language has become the industry standard for training of foundational AI models. The model’s weights are distributed across nodes, where different nodes handle different parts of the training process simultaneously, optimising efficiency and speed.
While other companies are catching up with NVIDIA, most of these are US-based. Amazon and Google are researching building their own GPUs to undercut NVIDIA. Another major challenge in GPU procurement is the restricted global supply.
Under the AI Diffusion Framework regulations, the US categorises countries into three tiers, each with specific restrictions on the export of AI chips and GPUs. Countries in the top tier have the least trade restrictions, whereas India, placed in the middle tier, faces stricter limits. These regulations cap exports at fewer than 1,700 GPUs per company annually to middle-tier countries.
While tech giants, such as Microsoft, scale their AI models by procuring nearly half a million GPUs from NVIDIA, Indian GPU providers, such as Yotta, operate on a much smaller scale, with an installed base of just 4,000 GPUs. Companies with larger GPU clusters can support more users and handle extensive workloads, benefitting from economies of scale that drive down computation costs through efficient workload distribution. “Currently, we offer GPU access at sub $3 per hour, significantly undercutting global prices of $25 per GPU per hour.
Our model prioritises high-volume adoption over high-margin sales. Instead of serving a limited customer base at premium prices, we aim to make AI computing accessible to a larger market with lower margins,” said Gupta. Mumbai-based data centre Neysa.
AI has taken a different route to bridge the domestic demand gap. It provides on-demand GPU infrastructure to create and deploy AI-based services to researchers and startups in the AI-based applications sector. They focus on efficient deployment of GPUs, ensuring maximum output with minimum number of units.
“We will always be a supply-constraint market. This is where players like us, deploy and make that GPU infrastructure available to the customers in the most efficient way possible,” said Sujit Janardanan, chief marketing officer at Neysa. While these GPU providers optimise resources and offer competitive pricing, their relatively small GPU clusters limit their ability to support large-scale AI workloads.
Microsoft procures over a million GPUs, while India’s Yotta has installed a base of just 4,000, though it plans to expand to 32,000. There still exists a vast gap. As demand for AI services surges, the gap in the domestic capacity of GPUs availability points toward the long-term challenge of achieving scalability in India’s AI ecosystem.
Also Read: A pick-me-up for India’s lithium dreams: How urban miners are spinning e-waste into white gold India focuses on domain-specific hardware rather than general purpose GPUs. While these hardware devices are tailored for specific workloads, the general-purpose GPUs are designed to handle heavy computational tasks efficiently, train a wide range of foundational models and ensure scalability in AI-based operations. “No full-fledged GPU has yet been designed indigenously.
India is still in the research and development and prototyping phase. Research is largely in AI accelerators and application-specific Integrated Circuits,” said Bibhudatta Sahoo, professor and head of computer science and engineering department at National Institute of Technology, Rourkela. Some of the IITs and research institutions in India have ventured into chip design, but it’s still at a nascent stage.
“The research in India remains limited to chip size of around 28nm to 65nm, whereas, at cutting-edge, global players, like Intel and TSMC, are now producing ultra-small chips of 10nm, 5nm, and even 2nm, to power the latest AI processors and provide high-performance computing devices,” said Sahoo. These chips form the backbone of GPUs and other advanced computing hardware. The term “nm” (nanometer) in the chip industry refers to the size of transistors in those chips.
As the transistor size decreases, more transistors can be accommodated into a given chip. This increases the computational power of the chip, thereby enhancing the performance and the efficiency of the GPU. The software used for designing and testing semiconductor chips is primarily developed and owned by US companies, such as Synopsys, Cadence and Mentor Graphics (owned by Siemens).
However, these tools come with high licensing costs, can be restricted under US export regulations, and might pose national security risks due to potential backdoor surveillance. This dependence not only limits access, but also threatens India’s long-term strategic autonomy in semiconductor innovation. “Some open-source softwares like OpenROAD, Magic, KLayout are being experimented with for chip designing.
However, they are not yet production-grade for advanced nodes. India needs to invest in indigenous Electronic Design and Automation toolchains as a strategic tech stack,” said Sahoo. The IndiaAI Mission is complemented by the Semicon India Programme launched in 2024, through which the government provides incentives to attract chip manufacturers and semiconductor firms, building foundations for GPU manufacturing in India.
“We have moved from being a complete absentee in the semiconductor landscape to now, today, slowly and systematically planting our flags and building our presence. I have absolutely no doubt that by the year 2026-27, we will have our first 28 nm fab in Gujarat,” former Union minister of state Rajeev Chandrashekhar had said last month at the 2nd Digital India RISC-V (DIR-V) Symposium at IIT Madras. The DIR-V initiative, launched in 2022, aims to develop homegrown semiconductor capabilities, which includes chip designing and reducing dependence on foreign technology.
One notable effort under this initiative is the Shakti processor project by IIT Madras. The premier technology institute has developed open-source RISC-V-based CPUs and is now exploring AI accelerators—specialised processors designed to enhance AI computations. This project will serve as a “step towards a GPU-like co-processor,” Sahoo said.
India will need 2.3 million AI professionals by 2027, but the country faces a projected shortfall of 1.2 million, according to a Bain & Company report released last month.
The required skillset includes managing large GPU infrastructure, AI workload optimisation, security and fault tolerance in multi-GPU environments. “A specialised talent pool is essential to manage this complex infrastructure effectively. Without it, inefficient deployment and potential damage to infrastructure could arise.
This specialised skillset is very difficult to find in the Indian market,” said Neysa.AI’s Janardanan. “India’s most powerful resource is its human capital.
Currently, this kind of training is limited to elite institutions. Scaling it up is critical.” Sneha Yadav is an intern with ThePrint (Edited by Mannat Chugh) Also Read: Surf’s up on Mars.
Red planet once had ‘vacation-style’ beaches, suggest new findings var ytflag = 0;var myListener = function() {document.removeEventListener('mousemove', myListener, false);lazyloadmyframes();};document.addEventListener('mousemove', myListener, false);window.
addEventListener('scroll', function() {if (ytflag == 0) {lazyloadmyframes();ytflag = 1;}});function lazyloadmyframes() {var ytv = document.getElementsByClassName("klazyiframe");for (var i = 0; i < ytv.length; i++) {ytv[i].
src = ytv[i].getAttribute('data-src');}} Save my name, email, and website in this browser for the next time I comment. Δ document.
getElementById( "ak_js_1" ).setAttribute( "value", ( new Date() ).getTime() );.
Technology
Lack of an indigenous GPU is a stumbling block to India’s AI ‘Atmanirbhar’ ambition. Here’s why
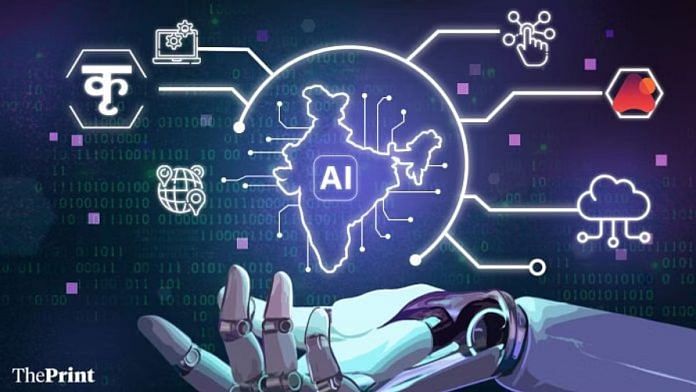
India remains dependent on foreign graphics processing units. As nations try to secure their own chip supply, GPUs have transformed from mere technology to a strategic asset.