Large Language Models (LLMs) have evolved far beyond their initial role as next-word predictors. Recent research, particularly from Anthropic, sheds light on the sophisticated mechanisms driving these systems, such as Claude. These findings reveal that LLMs are capable of reasoning, planning, and adapting in ways that challenge traditional assumptions about their functionality.
By exploring these advancements, we gain a deeper understanding of how LLMs process language and generate responses, paving the way for more effective and responsible applications. It turns out, LLMs operate in shared conceptual spaces that bridge languages, map out plans before generating text, and even blend rough approximations with precision when tackling math problems. But they’re not perfect—hallucinations, reasoning gaps, and vulnerabilities to clever prompts (or “jailbreaks”) highlight their limitations.
This overview by Prompt Engineering explores the fascinating mechanics behind LLMs, exploring how they think, where they falter, and what this means for their future applications. Let’s unpack the mystery of these digital minds together. LLMs operate within a shared conceptual space, allowing multilingual reasoning and cross-domain applications, making them versatile tools for global challenges.
Contrary to traditional views, LLMs plan responses beyond token-by-token generation, making sure coherence and adaptability in tasks like poetry or structured outputs. LLMs use a hybrid approach to mathematical reasoning, combining rough approximations with precise calculations, showcasing flexible problem-solving capabilities. Challenges like hallucinations, reasoning discrepancies, and jailbreak vulnerabilities highlight the need for improved transparency, reliability, and safety mechanisms in LLMs.
Neural circuit analysis provides insights into LLMs’ internal processes, enhancing interpretability and paving the way for more robust and accountable AI systems. LLMs operate within a shared conceptual space, a universal framework that enables them to map and understand concepts across multiple languages. This capability allows them to perform multilingual reasoning and translation with remarkable efficiency.
Larger models exhibit stronger alignment between languages, enhancing their ability to transfer knowledge across linguistic boundaries. This cross-linguistic capability is critical for solving problems in diverse contexts, where reasoning in one language can inform solutions in another. For instance, an LLM extensively trained in English can seamlessly apply its understanding to answer questions in Spanish or translate complex ideas.
This shared conceptual framework is not only foundational for multilingual applications but also positions LLMs as versatile tools for addressing global challenges. Contrary to the belief that LLMs generate text one word at a time, research reveals their ability to plan responses holistically. These models construct overarching frameworks before generating specific outputs, allowing them to adapt dynamically to various constraints.
When tasked with creating a poem, an LLM may first outline the structure, making sure coherence and stylistic consistency before filling in the details. In technical writing, the model can prioritize key points and organize information logically to meet specific requirements. This ability to plan and adjust dynamically demonstrates a level of sophistication that distinguishes LLMs from simpler predictive systems.
It also highlights their potential for applications requiring structured and coherent outputs, such as creative writing or technical documentation. Gain further expertise in Large Language Models (LLMs) by checking out these recommendations. LLMs approach mathematical reasoning through a hybrid method that combines rough approximations with precise calculations.
This dual approach allows them to solve problems adaptively, rather than relying solely on memorization or predefined algorithms. When performing addition, an LLM might first estimate the sum and then refine its answer through detailed reasoning. This flexibility enables the model to handle both simple calculations and complex problem-solving scenarios.
This adaptability is particularly valuable in fields requiring both speed and accuracy, such as data analysis or engineering. By using this hybrid approach, LLMs can address a wide range of technical and analytical challenges. One of the most intriguing aspects of LLMs is the gap between their reasoning chains and the actual internal processes they use to arrive at solutions.
While they often produce plausible explanations for their outputs, these explanations may not accurately reflect the underlying steps. LLMs sometimes skip intermediate steps or fabricate reasoning paths while still delivering correct results. This discrepancy raises questions about the interpretability and reliability of their outputs, particularly in high-stakes applications.
Understanding and addressing this gap is crucial for improving the transparency and trustworthiness of LLMs. By refining their reasoning processes, researchers can enhance the reliability of these systems, making sure they are better suited for critical tasks. Hallucination remains a persistent challenge for LLMs.
When faced with insufficient information, these models often refuse to answer. However, under certain conditions, they can generate fabricated responses that appear plausible but are factually incorrect. Misfiring neural circuits or overfamiliarity with incomplete data can lead to inaccurate outputs.
For example, an LLM might confidently provide an incorrect historical fact if it aligns with patterns encountered during training. Mitigating hallucination is essential for improving the reliability of LLMs, particularly in applications where accuracy is paramount, such as medical diagnostics or legal analysis. Ongoing research aims to address these issues by refining training processes and implementing more robust safeguards.
LLMs are susceptible to jailbreak attacks, where carefully crafted prompts bypass their safety protocols. These exploits take advantage of the tension between grammatical coherence and built-in safeguards, exposing vulnerabilities in the system. Embedding harmful instructions within otherwise benign queries to trick the model into generating unsafe responses.
Exploiting ambiguities in language to bypass ethical constraints. Strengthening safety mechanisms is critical to prevent misuse and ensure the ethical deployment of LLMs. By addressing these vulnerabilities, developers can create more secure systems that consistently uphold ethical standards.
Understanding how LLMs process inputs and generate outputs is a growing area of research. Neural circuit analysis provides valuable insights into the specific activations triggered by different inputs, offering a clearer picture of their internal mechanisms. Tracing how a particular question influences the model’s reasoning pathways.
Identifying areas where the model’s reasoning aligns or diverges from human logic. By uncovering the inner workings of LLMs, researchers can enhance their transparency and robustness. This knowledge not only builds trust in AI technologies but also informs the development of more reliable and accountable systems.
The advancements in LLMs have significant implications for their development and deployment. By understanding their reasoning, planning, and adaptability, you can address critical challenges and unlock their full potential. Refining training processes to improve accuracy and ethical alignment.
Implementing robust safety mechanisms to mitigate risks and prevent misuse. Enhancing transparency to build trust and accountability in AI systems. Balancing these advancements with responsible practices is essential for making sure that LLMs are both effective and ethical in their applications.
As research continues, these systems will play an increasingly important role in addressing complex, interdisciplinary challenges across the globe. Media Credit:.
Technology
How LLMs Are Redefining AI : Beyond Predicting the Next Word
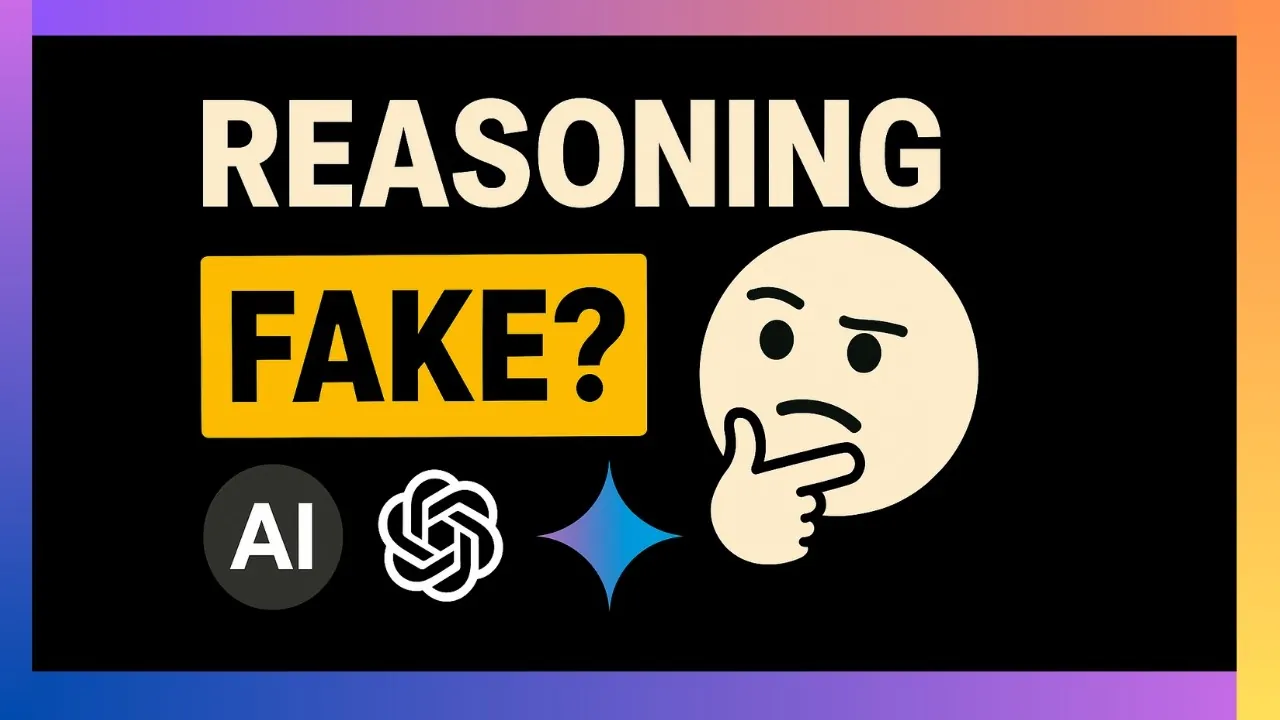
Large Language Models (LLMs) have evolved far beyond their initial role as next-word predictors. Recent research, particularly from Anthropic, sheds light on the sophisticated mechanisms driving these systems, such as Claude. These findings reveal that LLMs are capable of reasoning, planning, and adapting in ways that challenge traditional assumptions about their functionality. By exploring these [...]The post How LLMs Are Redefining AI : Beyond Predicting the Next Word appeared first on Geeky Gadgets.