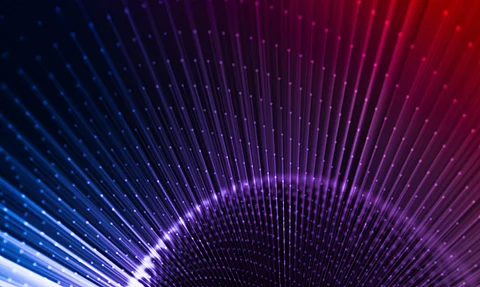
According to a recent AI survey by Dun & Bradstreet , 88% of organizations are implementing AI, but 54% of them express concerns over the quality and reliability of the data they are using. The effectiveness of AI, including its relevance and transparency, depends on the quality of the underlying data, and only 50% of organizations believe their data foundation is where it needs to be to implement AI properly. 64% of the organizations surveyed point out that automating tasks is the leading use case for their enterprise in terms of the best use cases for agentic AI, which involves AI agents working without constant human intervention.
42% report that enhancing human capacity is the main use case, followed by 36% for strengthening data management and 32% for analyzing market trends. Poor data quality derails AI projects The Rand Corporation reports that over 80% of AI projects fail , which is twice as many as IT projects that don’t use AI. Common causes include data issues, misunderstanding of the problem, deficient infrastructure, technology focus, and problem complexity.
Data quality is a critical factor because AI projects, in contrast to traditional ones involving app development, are essentially data integration projects and merit a corresponding approach. The problems with data quality aren’t new. Businesses have been struggling with it for decades, investing great efforts and funds to address the challenges.
Poor data quality costs organizations trillions every year. These losses draw attention to an ongoing struggle to maintain quality and the significant financial consequences of failure to do so. Poor data quality manifests in inconsistencies, inaccuracies, incompleteness, and other issues, which can disrupt AI projects in many ways.
Projects cost more and take longer as people spend excessive time cleaning and validating data. Poor data obstructs AI solutions’ scalability, limiting their reach and effectiveness. AI models trained on erroneous data generate unreliable outputs, leading to flawed strategies and decisions.
Finally, quality issues compromise confidence in AI initiatives, potentially making it more challenging to secure investments. AI agents compete for rewards to improve data quality A possible solution is pioneered by Fraction AI , a protocol that builds on AI data generation. It involves competition between AI agents to generate quality data while earning rewards.
Essentially, AI agents battle to generate the best outputs, with economic incentives promoting consistent improvement. Fraction AI brings builders and stakers into a symbiotic relationship. Builders use simple prompts to create and launch AI agents.
No coding is required to build the agents that compete in data generation tasks. Top-performing agents earn rewards from the competition pool. Stakers stake ether as the protocol’s economic foundation.
They earn consistent yields through data licensing revenue, protocol fees, competition fees, etc. Staking enables builders to receive rewards and helps secure Fraction AI. Five agents compete to produce the highest-quality data every minute.
The prize pool is created through participation fees, which each agent pays. Five agents are chosen to face off in each round, and they have a minute to generate data based on the respective task. AI validation helps assess outputs for quality.
The best performers are rewarded, with a direct relationship between data quality and returns. The protocol also uses historical track records and format compliance to evaluate quality in real-time. Continuous improvement is ensured by adjusting quality standards based on overall real-time ecosystem performance.
Robust data governance practices are another possible solution to mitigate challenges and enhance AI project success rates. Organizations should develop and track metrics to gauge data quality, such as timeliness, completeness, and accuracy. Regular audits can help address issues proactively.
Specific individuals within the organization should be responsible for data quality to ensure accountability and continuous data standard monitoring. Other measures include investing in data-cleaning tools, using external expertise, or fostering a data-driven culture. Advanced preprocessing and cleaning tools automate data anomaly detection and correction.
Data quality consultants can provide insights tailored to the specific industry and its unique challenges, and training programs can be put in place to encourage employees to grasp the importance of data quality and begin to value it. Other concerns about AI implementation cited by survey respondents include data security, privacy violations, and disclosure of sensitive or proprietary information, with 46%, 43%, and 42% naming them. The companies are at different stages of implementation, including piloting programs or products (10%), developing AI products (24%), deploying AI solutions (25%), and exploration and research (29%).
Companies face two critical roadblocks as they integrate AI across functions: navigating regulatory and ethical challenges and access to trusted business data. 33% of organizations point to each of these roadblocks. Regardless of their position in their AI implementation journey, companies express difficulties with aligning on business priorities (31%) and internal expertise (31%), explaining and interpreting the technology (28%), assessing the risks (27%), showcasing returns (25%), and achieving AI transparency (25%) According to 42% of businesses currently deploying AI, the highest level of progress has come from streamlining processes, followed by 39% with co-piloting, 38% with supplementing current tasks, 21% with KPIs and measurement, 18% with modeling scenarios, and 13% with eliminating personnel bias.
In response to the question about which AI trends will have the biggest impact on businesses in 2025, 51% of organizations cite intelligent automation, followed by 46% with conversational AI, 33% with visual AI and multimodality, and 23% with hyper-personalized marketing. Around 25% say they are preparing for the effects of new compliance and governance frameworks..