Share Share Share Share Email Artificial intelligence (AI) has transformed financial services, enabling faster decision-making in credit scoring, loan approvals, fraud detection, and risk assessments. However, as these technologies become more embedded in financial systems, concerns about algorithmic bias have grown. In their recent work, Rahul Vats and co-author Shekhar Agrawal and Srinivasa Sunil Chippada introduce a groundbreaking Bias Detection and Fairness Evaluation (BDFE) Framework to tackle this issue.
This article explores how this innovation is shaping the future of AI-driven financial services. The Challenge of Algorithmic Bias in Finance AI models in finance can perpetuate historical biases, creating a cycle of discrimination. When deployed in lending decisions, these systems disproportionately deny minority applicants and charge them higher interest rates when approved.
Similar disparities appear in insurance claim processing times and approval rates across demographic groups. These automated inequities compound over generations, creating widening wealth gaps and eroding consumer confidence in financial institutions. Without proactive debiasing strategies and regular equity audits, algorithmic discrimination becomes more entrenched and difficult to reverse, particularly as financial services increasingly rely on automated decision-making systems for efficiency gains.
Introducing the BDFE Framework The BDFE Framework provides a structured approach to detecting and mitigating AI bias in financial services. It combines adversarial testing, fairness-aware training, and explainable AI techniques to identify and reduce bias while maintaining model accuracy and regulatory compliance. Unlike traditional fairness interventions, this framework accounts for complex bias patterns, ensuring a more equitable application of AI in finance.
Adversarial Testing: Uncovering Hidden Bias One of the standout features of the BDFE Framework is its adversarial testing methodology. By systematically generating inputs that vary only by protected attributes (such as race or gender), researchers can detect disparities in AI decision-making. This technique is particularly effective in financial applications, where seemingly neutral data points like ZIP codes or education history can act as proxies for sensitive demographic factors.
These proxy variables perpetuate historical patterns of discrimination unintentionally. Fairness-Aware Training and Optimization The framework employs fairness-aware training methods to proactively reduce bias during AI model development. Techniques such as adversarial debiasing ensure that AI systems optimize both accuracy and fairness.
Additionally, the framework integrates fairness constraints within the training process, preventing the reinforcement of discriminatory patterns. Explainable AI for Regulatory Compliance A major barrier to fair AI adoption in finance is the lack of transparency in decision-making processes. The BDFE Framework addresses this challenge by incorporating explainable AI (XAI) techniques.
Feature attribution methods like SHAP values and attention visualization help financial institutions understand why certain decisions are made, ensuring compliance with regulatory requirements such as the Equal Credit Opportunity Act (ECOA) and the General Data Protection Regulation (GDPR). Continuous Monitoring for Bias Prevention Bias in AI models can evolve over time due to changing data distributions. The BDFE Framework includes continuous monitoring protocols that track fairness metrics throughout an AI system’s lifecycle.
By implementing automated fairness dashboards and real-time alerts, financial institutions can detect emerging biases before they impact customers. Real-World Benefits of Fair AI Beyond regulatory compliance, fairness-enhanced AI systems offer significant business advantages. By reducing bias, financial institutions can expand their customer base, decrease litigation risks, and improve customer trust.
The implementation of bias-aware AI models has already led to measurable improvements in credit decisioning and fraud detection accuracy while maintaining fairness across demographic groups. The Future of Fair AI in Financial Services As AI continues to shape financial decision-making, frameworks like BDFE will become essential for ensuring ethical and unbiased outcomes. Future advancements in fairness-aware AI will likely include self-correcting models that adapt to evolving fairness standards, as well as collaborative industry-wide efforts to establish common bias detection protocols.
In Conclusion, Rahul Vats and his co-author have introduced a pioneering approach to addressing bias in financial AI. The BDFE Framework provides financial institutions with the tools needed to create fairer, more transparent AI models. By integrating adversarial testing, fairness-aware training, explainable AI, and continuous monitoring, this framework sets a new standard for ethical AI deployment in financial services.
As institutions adopt these principles, the financial industry will move closer to a more inclusive and equitable future. Related Items: AI , Bias Detection , Rahul Vats Share Share Share Share Email Recommended for you Revolutionizing Healthcare with Cloud-Native Multimodal AI Systems Enhancing Trading Efficiency Through Human-AI Collaboration AI-Driven Performance and Chaos Engineering: Reshaping Cloud-Native Architectures Comments.
Technology
Ensuring Fairness in Financial AI: A New Approach to Bias Detection
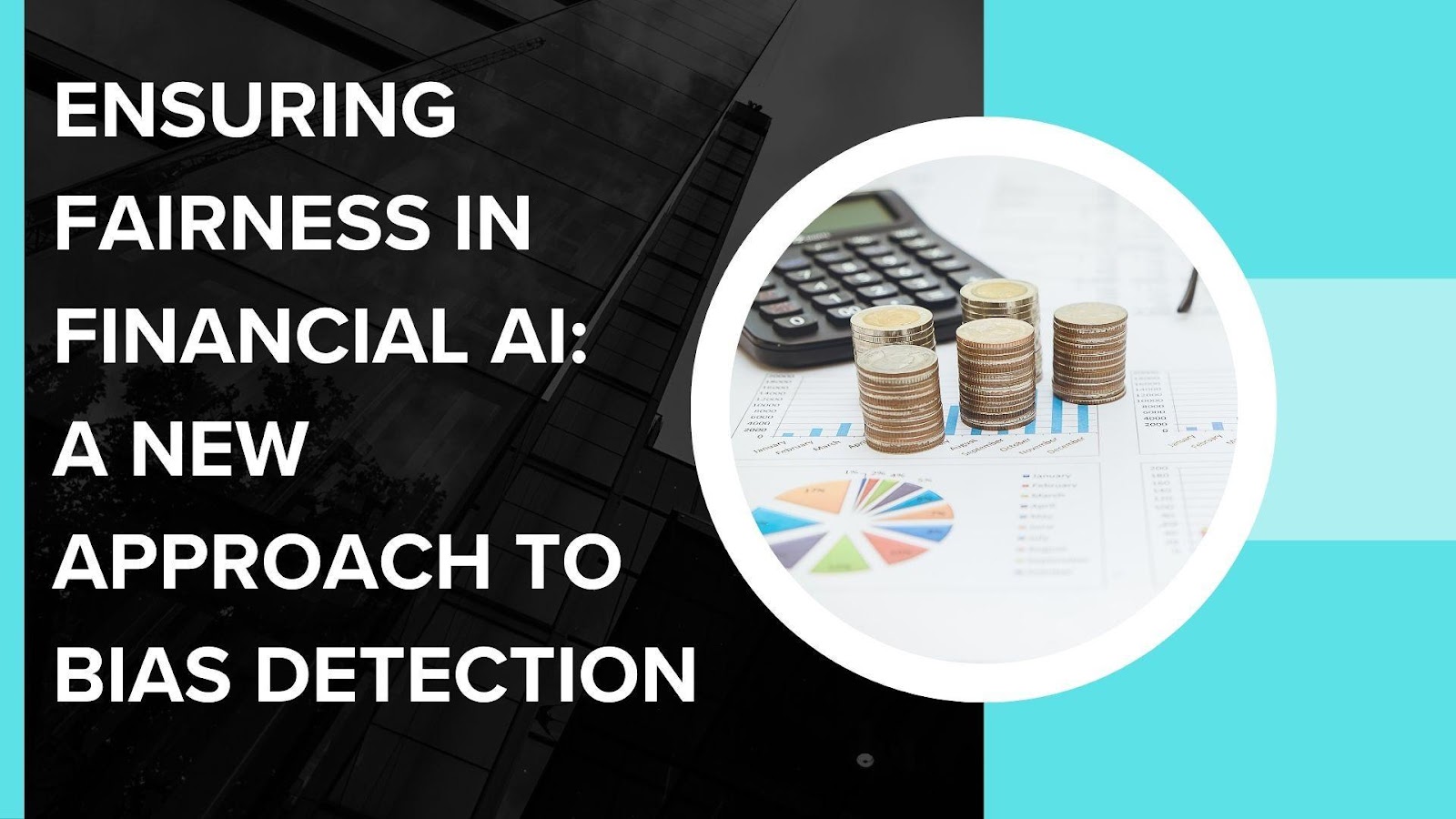
Artificial intelligence (AI) has transformed financial services, enabling faster decision-making in credit scoring, loan approvals, fraud detection, and risk assessments. However, as these technologies become more embedded in financial systems, concerns about algorithmic bias have grown. In their recent work, Rahul Vats and co-author Shekhar Agrawal and Srinivasa Sunil Chippada introduce a groundbreaking Bias Detection [...]The post Ensuring Fairness in Financial AI: A New Approach to Bias Detection appeared first on TechBullion.