Researchers from top US universities warn extending pre-training can be detrimental to performance Too much pre-training can deliver worse performance due to something akin to the butterfly effect The more they are pre-trained, the more they become sensitive to small changes that could disrupt the end result Researchers from Carnegie Mellon, Stanford, Harvard, and Princeton are challenging one of AI development’s accepted core beliefs - that the more pre-training data the better the performance. As reported by HPCwire , a new paper discuses the concept of “catastrophic overtraining,” whereby extended pre-training can harm a model’s performance after fine-tuning. The researchers compared two versions of the OLMo-1B model, one trained on 2.
3 trillion tokens and another on 3 trillion. Despite the larger training set, the more extensively trained model reportedly performed up to 3% worse on benchmarks like AlpacaEval and ARC. DeepSeek and the race to surpass human intelligence Shut it all down? Microsoft research suggests AI usage is making us feel dumber – but you don't need to panic yet Reaching the inflection point This performance drop, the study claims, is linked to a phenomenon called “progressive sensitivity.
” As the token count increases, the model becomes more fragile. Even small tweaks, like adjustments during fine-tuning, or the introduction of noise, can reverse earlier gains. The authors demonstrated this by injecting Gaussian noise into pre-trained models, noting that performance degraded more sharply the longer the model was trained.
The point where this additional training starts to degrade performance is called the “inflection point.” Are you a pro? Subscribe to our newsletter Sign up to the TechRadar Pro newsletter to get all the top news, opinion, features and guidance your business needs to succeed! Once reached, the benefits of training start to become outweighed by the risk of internal instability. The study found that this tipping point often occurs beyond 2.
5 trillion tokens in smaller models, like OLMo-1B. “Catastrophic overtraining may be inevitable..
. especially when the pre-training and fine-tuning tasks are misaligned,” the authors warn in their paper, which you can access through the arXiv pre-print server . While the researchers are not suggesting an end to pre-training, they do feel that developers should consider just how much pre-training is enough.
As the paper concludes, “Our findings call for a renewed focus on model scaling that considers the entire training pipeline.” For AI developers chasing scale, the message seems clear: sometimes, less really is more. 'An extension of a scientist's brain': Researchers explore AI to augment inspiration Researchers design tech that could 'potentially replace solar cells' in applications A new AI tool wants to make serendipitous scientific discovery less human.
Technology
'Catastrophic overtraining' could harm large language AI models that are trained on more data for the sake of training
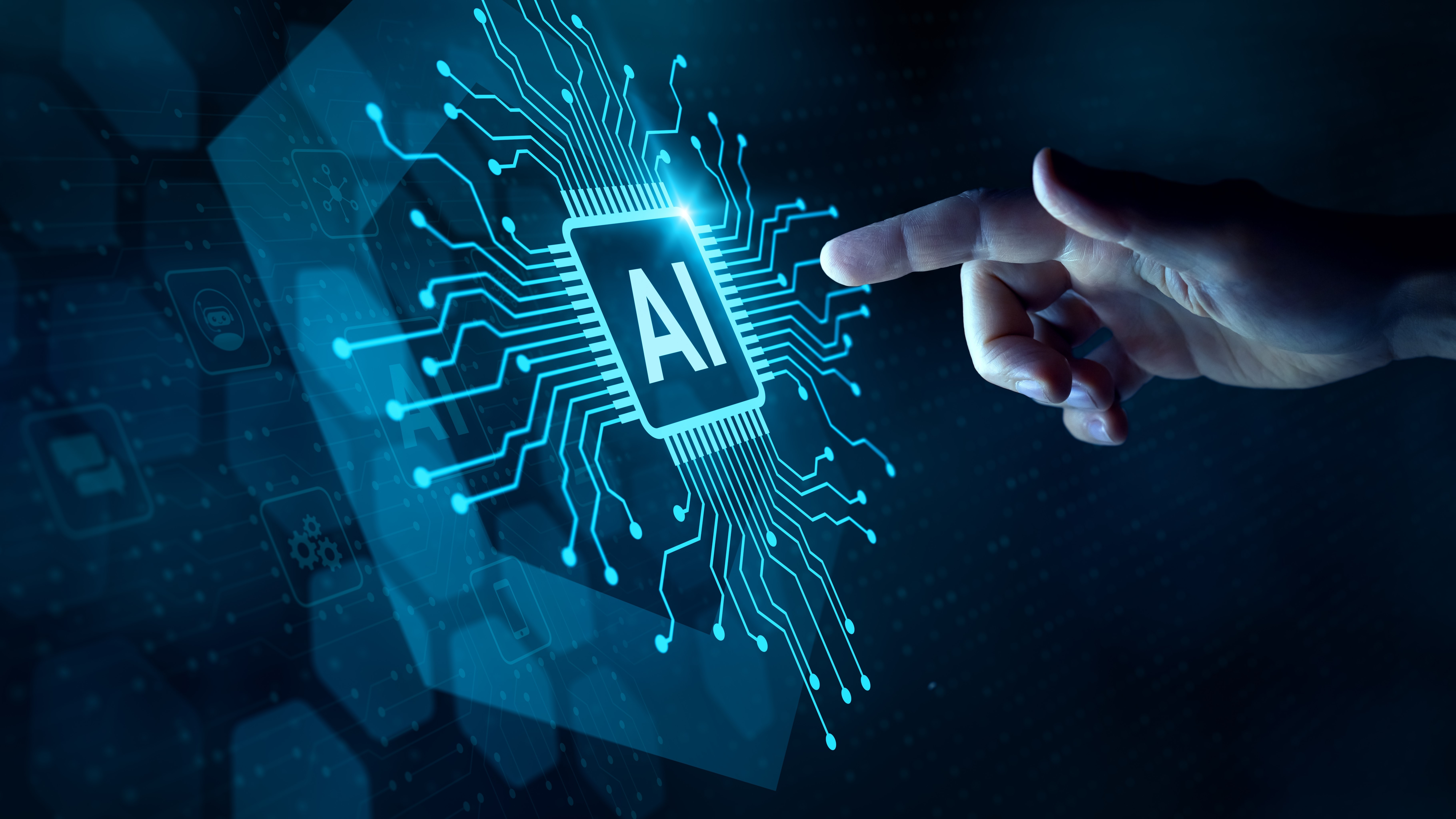
University researchers found that over training large language AI models resulted in worse performance.