The is reshaping how AI agents interact with tools and services. By introducing a centralized and dynamic toolbox, mCP eliminates the need for manual configurations, allowing agents to seamlessly discover, select, and execute tools. This approach detailed by Neil Stephenson significantly reduces complexity, optimizes setup time, and enhances workflow efficiency, making it a pivotal advancement for modern AI applications.
mCP (Model Context Protocol) simplifies AI agent interactions by providing a centralized, dynamic toolbox, eliminating manual configurations and streamlining workflows. Unlike traditional setups, mCP dynamically connects agents to tools via a centralized server, reducing complexity, shortening prompts, and making sure immediate tool access. Key benefits of mCP include reduced setup complexity, streamlined workflows, and enhanced efficiency for AI-driven processes.
Implementation involves setting up a self-hosted n8n platform, configuring API keys, and accessing tools through GitHub repositories for flexibility and security. While mCP is ideal for prototyping and dynamic workflows, users should consider potential stability and security risks when integrating third-party servers. mCP is a protocol designed to simplify and enhance the interaction between AI agents and external tools or services.
It operates through a centralized server that dynamically provides tool descriptions and schemas. This eliminates the need for manually configuring each tool or crafting detailed prompts, allowing agents to function more effectively. With mCP, you can focus on designing and refining workflows without being hindered by integration challenges.
By centralizing tool management, mCP ensures that AI agents can access the resources they need without unnecessary delays or complications. This streamlined approach allows developers to allocate more time to innovation and less to troubleshooting. Traditional AI agent setups often require extensive manual effort.
Developers must individually describe, configure, and integrate each tool, which can be both time-consuming and error-prone. mCP offers a fantastic alternative by connecting agents to a centralized server that dynamically provides all necessary tool information. This approach introduces several key improvements: The centralized server eliminates the need for manual configurations, simplifying the integration process.
Agents receive tool information dynamically, reducing the need for detailed, tool-specific prompts. Agents can quickly discover and use the tools they need, enhancing overall efficiency. These features make mCP a more efficient and reliable solution for integrating tools into AI workflows, addressing many of the challenges associated with traditional setups.
Here is a selection of other guides from our extensive library of content you may find of interest on . Adopting mCP offers a range of advantages that can significantly enhance AI-driven processes: Centralized tool management minimizes the need for manual configurations, lightening the workload for developers. Agents dynamically receive tool information, eliminating the need for lengthy and detailed prompts.
Tools are easily discoverable and executable, allowing you to focus on optimizing workflows rather than resolving integration issues. The dynamic nature of mCP enables rapid adjustments and scalability as workflows evolve. These benefits position mCP as an essential protocol for developers and organizations aiming to improve the efficiency and adaptability of their AI systems.
Implementing mCP is a straightforward process that involves setting up a self-hosted version of the n8n automation platform and integrating relevant community nodes. Follow these steps to get started: Deploy n8n on a self-hosted server to maintain full control over your workflows and ensure data security. Securely connect to mCP servers by setting up API keys and credentials to enable seamless integration.
Explore GitHub repositories for official and third-party integrations to expand your toolbox and enhance functionality. This setup creates a robust environment where AI agents can operate efficiently while maintaining flexibility and security. By using mCP, you can build workflows that are both dynamic and reliable.
mCP is particularly well-suited for experimental and developmental AI workflows. Its ability to enable rapid prototyping and testing with minimal configuration makes it ideal for: Quickly create and refine AI agents without being bogged down by integration challenges. Experiment with new tools and workflows to drive innovation in AI applications.
However, there are certain limitations to consider when using mCP: mCP may encounter stability issues in production environments, particularly when relying on third-party servers. Integrating external services through third-party servers can introduce vulnerabilities, requiring careful evaluation and monitoring. Despite these challenges, mCP remains a valuable tool for simplifying AI workflows and enhancing the capabilities of AI agents.
The potential of mCP is immense. As more servers and tools become available, the protocol is expected to evolve, further simplifying tool integration and improving workflow efficiency. These advancements will likely solidify mCP’s role as a cornerstone of AI development, allowing the creation of more powerful and adaptable AI agents.
Future developments may include enhanced security measures, broader compatibility with diverse tools, and improved stability for production environments. These improvements will ensure that mCP continues to meet the growing demands of AI-driven industries. When using mCP, it is essential to approach its implementation thoughtfully to maximize its benefits while minimizing potential risks.
Key considerations include: Assess the reliability and security of third-party servers before integrating them into your workflows to avoid vulnerabilities. Use guides, documentation, and community support to ensure a smooth and efficient setup process. Regularly review and optimize your workflows to address any stability or performance issues that may arise.
By taking these precautions, you can fully harness the capabilities of mCP while making sure the security and reliability of your AI systems. Media Credit:.
Technology
Build mCP n8n Supercharged AI Agents in Just 10 mins
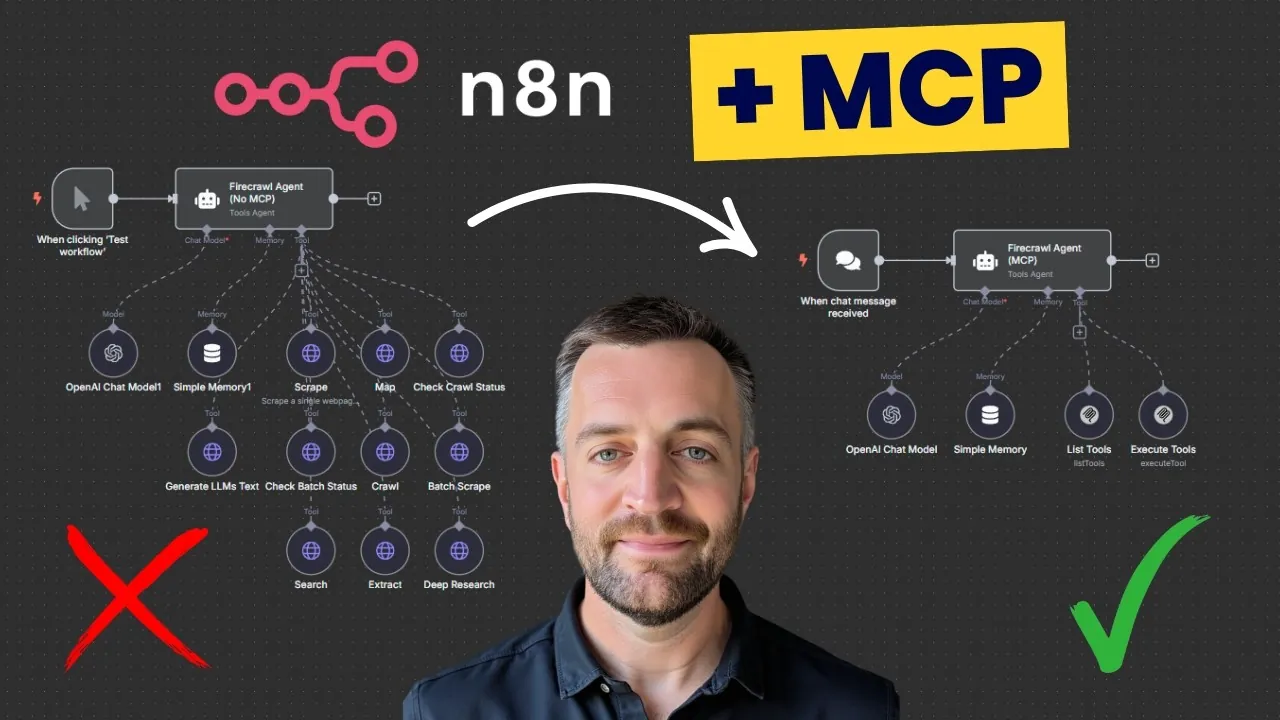
The Model Context Protocol (mCP) is reshaping how AI agents interact with tools and services. By introducing a centralized and dynamic toolbox, mCP eliminates the need for manual configurations, allowing agents to seamlessly discover, select, and execute tools. This approach detailed by Neil Stephenson significantly reduces complexity, optimizes setup time, and enhances workflow efficiency, making [...]The post Build mCP n8n Supercharged AI Agents in Just 10 mins appeared first on Geeky Gadgets.