Share Share Share Share Email Artificial Intelligence (AI) has made remarkable strides, but its decision-making processes often remain a mystery. In her exploration of Explainable AI (XAI) for large-scale predictive systems, Priyadharshini Krishnamurthy highlights the latest advancements in AI transparency. This article delves into groundbreaking innovations that enhance model interpretability while balancing accuracy, efficiency, and trust.
The Evolution of Explainable AI The need for AI interpretability has grown alongside its widespread adoption. Initially, rule-based systems provided clear reasoning for decisions, but as AI evolved into deep learning and complex predictive models, explanations became more elusive. Modern XAI techniques seek to restore interpretability by offering methods that explain AI’s inner workings in a way that stakeholders can understand.
Model-Agnostic Techniques: Interpreting Any AI System One major innovation in XAI is the development of model-agnostic techniques—methods that can be applied regardless of the AI model in use. Feature Importance Methods highlight which variables most influence a model’s predictions. These methods have evolved to include real-time monitoring, ensuring that as data changes, so do the insights into what drives AI decisions.
Partial Dependence Plots (PDPs) have also seen improvements. While traditional PDPs could misinterpret correlations, new versions incorporate confidence bands and cluster analyses to provide more accurate visual explanations of AI predictions. SHAP (SHapley Additive explanations) values offer a mathematically sound approach to explaining AI outputs by distributing credit across input features.
Innovations like TreeSHAP and DeepSHAP optimize this process for tree-based and deep learning models, respectively, making AI explanations faster and more accurate. LIME (Local Interpretable Model-agnostic Explanations) has also undergone enhancements, addressing stability concerns by refining how it selects local data samples for explanation. The introduction of hierarchical LIME now allows for multi-layered insights into AI decisions, providing granular explanations based on user needs.
Counterfactual Explanations , which provide alternative scenarios showing how an AI decision would change with different inputs, have evolved with improved optimization techniques. These methods now generate more realistic, domain-specific counterfactuals that align with real-world constraints. Model-Specific Approaches: Deep Diving into AI Architecture Neural Network Visualization methods have improved significantly, particularly in understanding how deep learning models process information.
Techniques such as Layer-wise Relevance Propagation (LRP) and integrated gradients now reveal how different layers contribute to the final AI decision, enhancing transparency in applications like image and speech recognition. Attention Mechanisms are a staple of modern deep learning, particularly in natural language processing. Recent advances enable these mechanisms to visualize how AI assigns importance to different input components, making AI’s reasoning process clearer.
Rule Extraction Methods , which translate deep learning predictions into human-readable rules, have been enhanced through fuzzy logic and symbolic reasoning. These techniques bridge the gap between AI complexity and human comprehension, particularly in fields that demand clear accountability, such as healthcare and finance. Explainable AI in Action: Enhancing Trust Across Industries Explainable AI is transforming industries where transparency is non-negotiable.
In healthcare, AI is now assisting doctors by providing layered explanations of medical diagnoses, breaking down decisions into digestible insights for both medical professionals and patients. Medical imaging AI, for instance, can highlight which features in an X-ray influenced its diagnostic conclusion, fostering greater trust in automated healthcare solutions. This crucial advancement allows practitioners to verify reasoning pathways and ensure appropriate treatment recommendations.
Challenges and Future Directions in Explainability Despite these advancements, Explainable AI still faces hurdles. Scalability remains a significant issue, as real-time explanations in large-scale AI systems require immense computational power. Researchers are addressing this by developing hierarchical explanation architectures that dynamically adjust explanation detail based on system load.
Interpretation complexity also poses a challenge. Users often struggle to make sense of highly technical AI explanations. To tackle this, adaptive explanation interfaces are being developed, allowing users to interactively explore AI insights at different levels of detail.
In conclusion, as AI continues to influence various aspects of society, ensuring its decisions are transparent and trustworthy is crucial. Priyadharshini Krishnamurthy, highlights the significance of XAI in developing responsible AI systems that emphasize clarity and accountability. Future advancements will focus on enhancing scalable, user-friendly, and ethically driven explanation techniques, ensuring AI remains both effective and understandable in the years ahead.
Related Items: Artificial intelligence , Priyadharshini Krishnamurthy Share Share Share Share Email Recommended for you The AI Revolution in Financial Risk Management and Predictive Analytics AI Innovation Hotspots: Top Cities Pioneering Artificial Intelligence in 2025 The Revolution of Artificial Intelligence and Blockchain in the Financial and Agricultural Industries Comments.
Technology
Bringing Transparency to AI: Innovations in Explainability
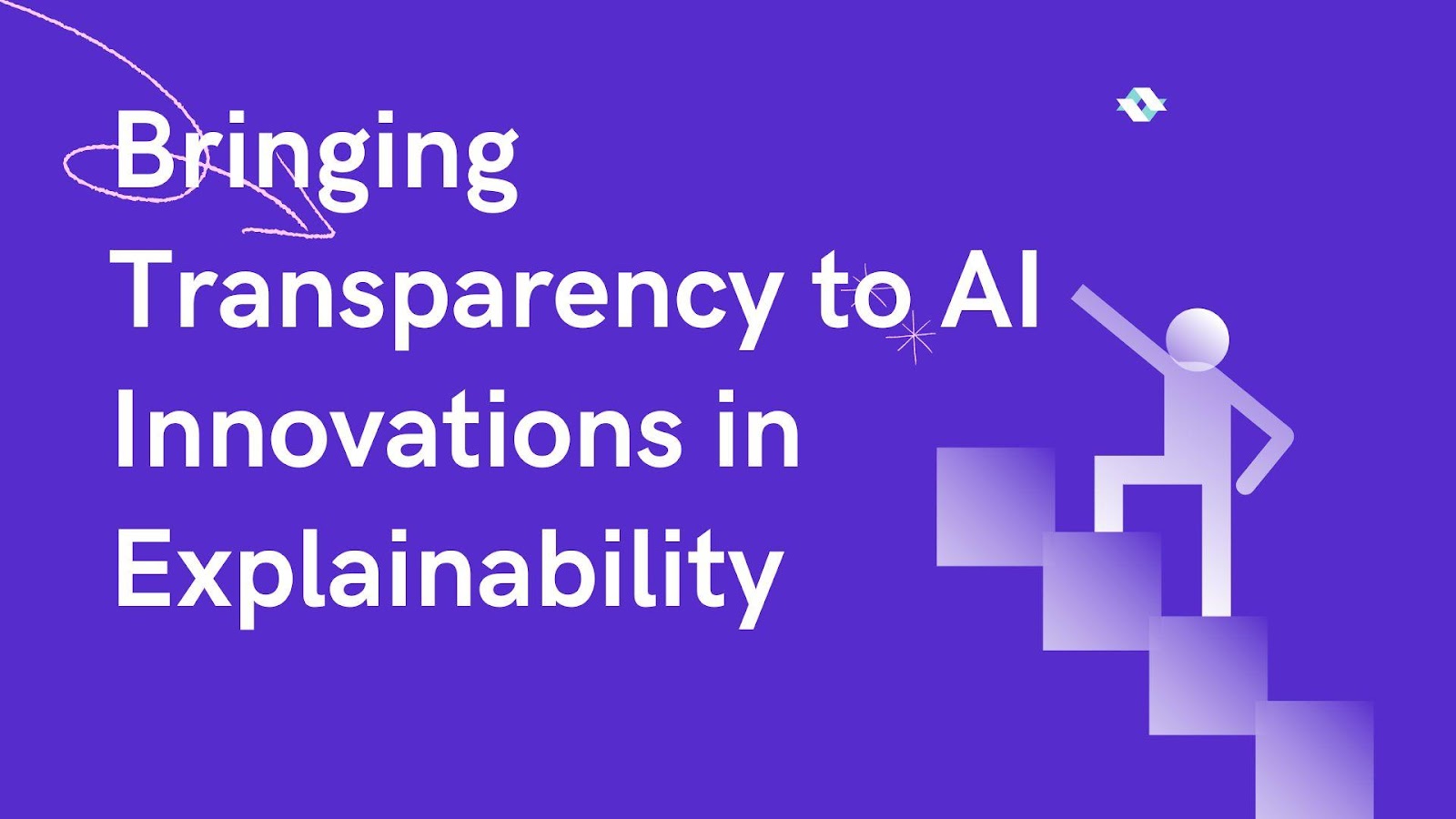
Artificial Intelligence (AI) has made remarkable strides, but its decision-making processes often remain a mystery. In her exploration of Explainable AI (XAI) for large-scale predictive systems, Priyadharshini Krishnamurthy highlights the latest advancements in AI transparency. This article delves into groundbreaking innovations that enhance model interpretability while balancing accuracy, efficiency, and trust. The Evolution of Explainable [...]The post Bringing Transparency to AI: Innovations in Explainability appeared first on TechBullion.