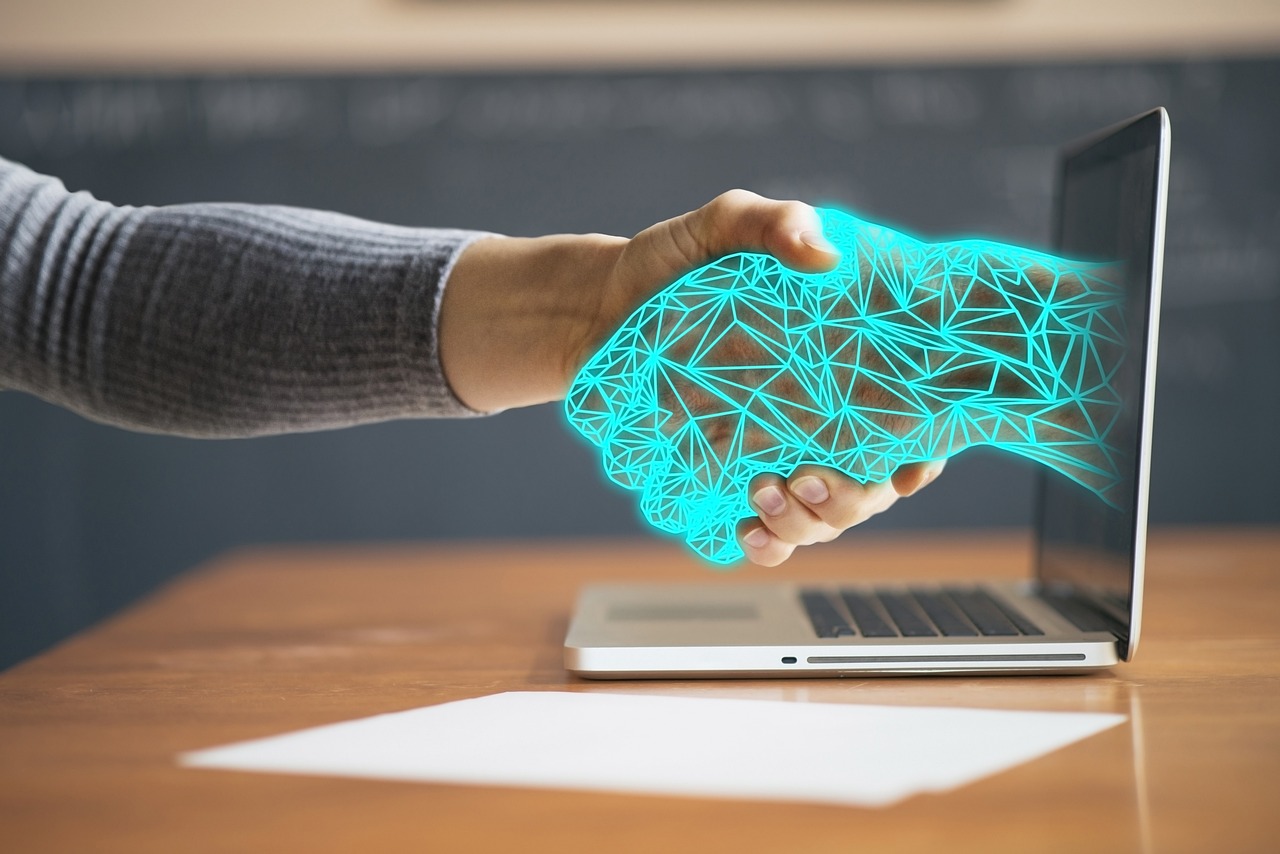
Share Tweet Share Share Email The Double-Edged Sword of AI Innovation With AI adoption, businesses gain three key advantages of operational efficiency together with predictive analytics and customer-specific interactions. The majority of 70% AI projects fail to achieve their goals mainly because of three common errors which include bad data quality alongside wrong strategic direction and insufficient governance. Advancing sustainable AI integration goes beyond obtaining advanced tools since it needs specific AI plans along with strategic management to handle risks and maximize value potential.
Common AI Pitfalls and Their Hidden Costs Organizations often stumble at three critical junctures: Data Disarray: AI accuracy suffers failure before execution because of insufficient or disconnected data sources. Misaligned Priorities: Organizations pursue superficial AI uses that neglect their essential business targets. Scalability Shortfalls: The success of pilot projects in managed conditions leads to their failure during large-scale implementation.
An early approach to solve these issues creates a path to successful outcomes while avoiding expensive path deviations. Phase 1: Mapping the Terrain – Assessing Organizational Readiness A successful AI roadmap begins with assessment activities. Leaders must evaluate: Data Health: Quality, accessibility, and governance maturity.
Infrastructure: Compatibility with AI workloads (cloud, on-premises, or hybrid). Workforce Skills: Gaps in technical expertise and change management readiness. The audit exposes vulnerabilities because it ignores neither simple improvements that enable task automation nor major enhancements for customer service chatbots.
Phase 2: Strategic Alignment – Bridging AI and Business Goals The business transformation consultant performs a critical function by interpreting technical capabilities for strategic business needs. Key steps include: Use Case Prioritization: Organizations should concentrate efforts on maximizing results from initiatives that focus on supply chain improvements and predictive equipment maintenance. ROI Forecasting: The organization needs to find a balance between immediate cost-cutting benefits and sustained customer loyalty.
Ethical Guardrails: Corporate entities need to introduce standards that protect data privacy along with methods to reduce bias and regulations to follow. The final stage makes sure that AI implementations create direct value for generating more revenue or making operations stronger. Phase 3: Building a Scalable Foundation Sustainability hinges on two pillars: Governance Frameworks: The implementation requires policies which ensure data integrity and model monitoring together with accountability standards.
Modular Infrastructure: The implementation of cloud-native platforms helps organizations achieve better need adaptation while ensuring they keep options open for switching vendors. The retailer implements AI for inventory management at the start but develops future systems for personalized marketing after establishing original infrastructure. Phase 4: Iterative Implementation – Test, Learn, Scale Rather than “big bang” rollouts, agile implementation minimizes risk: Proof of Concept (PoC): Validate AI’s feasibility in a controlled environment.
Pilot Launch: Measure performance against KPIs like error rates or user adoption. Phased Scaling: Expand successful pilots while continuously refining models. The testing of AI-driven diagnostics by a healthcare provider begins in single clinics as a preliminary step before extending to complete organization-wide deployment.
The Human Factor: Upskilling and Change Management Technology alone can’t drive transformation. Organizations must: Reskill Teams: Train employees on AI tools and data literacy. Foster Collaboration: Break down silos between IT, data scientists, and business units.
Communicate Transparently: Address workforce anxieties about AI’s role in workflows. Business transformation consultants serve as cultural shift facilitators through their work to make teams understand that AI acts as an enhancement rather than posing threats to their current responsibilities. Why Expertise Matters: The Consultant Advantage? Navigating AI integration requires more than technical know-how.
A seasoned business transformation consultant offers: Objective Insights: Unbiased evaluation of internal capabilities and market trends. Cross-Industry Wisdom: Lessons from successful (and failed) AI deployments in similar sectors. Risk Mitigation: Proactive identification of legal, financial, or operational blind spots.
The outside view of consultants enables companies to prevent short-sighted choices that strike a balance between making progress through innovation and staying practical. Conclusion: Future-Proofing Through Foresight Sustainable AI integration isn’t about chasing the latest algorithms—it’s about building adaptable systems, aligned strategies, and a culture ready for continuous evolution. By adopting a structured AI roadmap and leveraging expert guidance, businesses can sidestep common pitfalls while positioning themselves as industry pioneers.
In an era where AI’s potential is limitless but its pitfalls are pervasive, preparedness is the ultimate competitive edge. Related Items: AI , Tech Integration Share Tweet Share Share Email Recommended for you Innovative AI Solutions in Real-Time Fraud Detection Revolutionizing Hyperscale Data Center Management: Integrating AI, AR, and Computer Vision AI-Driven Predictive Analytics: Transforming Enterprise Systems Comments.