Cybersecurity is undergoing a major transformation with artificial intelligence (AI) playing a pivotal role in identifying and preventing cyber threats . Dinesh Rajasekharan , an expert in AI-driven analytics, explores how advanced machine learning techniques are reshaping cybersecurity. His research highlights innovations that enhance threat detection, making digital security more proactive and adaptive.
The Shift from Reactive to Proactive Security Traditional cybersecurity methods relied on reactive approaches, detecting threats only after they had infiltrated systems. AI, however, has shifted this paradigm by enabling predictive security measures. Through machine learning algorithms, AI identifies anomalies and patterns in vast datasets, allowing cybersecurity systems to detect threats before they materialize.
This proactive approach significantly reduces response times, preventing potential breaches. Anomaly Detection: Recognizing Unusual Behavior One of AI's most significant contributions to cybersecurity is anomaly detection. AI models analyze normal system behavior and flag any deviations that may indicate an attack.
Unlike traditional security systems that depend on known threat signatures, AI-powered anomaly detection can identify previously unseen cyber threats, including zero-day vulnerabilities. This capability enables rapid identification of intrusion attempts, malware propagation, and data exfiltration in real-time. Machine learning algorithms continuously adapt to evolving network conditions, reducing false positives while maintaining vigilance.
Advanced neural networks can detect subtle behavioral patterns that human analysts might miss, providing organizations with critical response time during emerging attacks. Pattern Recognition for Enhanced Security Pattern recognition enables AI to analyze large volumes of network traffic, identifying suspicious activity. By continuously learning from past incidents, AI improves its accuracy in detecting sophisticated cyberattacks.
This ability helps prevent data breaches and unauthorized access by recognizing behavior that deviates from established security norms. Real-Time Threat Intelligence: A Game Changer AI's capability to provide real-time threat intelligence is revolutionizing cybersecurity. AI systems monitor network activity 24/7, analyzing data from multiple sources to detect and mitigate threats as they emerge.
This immediate response prevents attackers from causing significant damage, ensuring business continuity and data protection. These intelligent systems can correlate seemingly unrelated events across disparate network segments, revealing coordinated attack campaigns that traditional tools would miss. By leveraging natural language processing, AI analyzes threat intelligence feeds from global sources, contextualizing emerging threats against an organization's specific vulnerabilities.
Challenges and Ethical Considerations Despite AI's advancements, challenges remain. False positives and negatives in AI-driven cybersecurity can impact security decisions. Additionally, ethical concerns, such as data privacy and AI bias, require careful regulation.
Addressing these issues is crucial to ensuring AI-driven cybersecurity remains reliable and fair. Security teams must contend with alert fatigue from excessive false alarms, while sophisticated attackers increasingly develop adversarial techniques to evade detection. Implementation costs and technical complexity create barriers for smaller organizations.
The shortage of skilled professionals who understand both cybersecurity and AI compounds these challenges. Moreover, as threat actors weaponize AI for attacks, defenders face an escalating technological arms race that demands continuous innovation and cross-industry collaboration..
Towards Autonomous Cybersecurity Systems The ultimate goal of AI in cybersecurity is to develop autonomous security systems that can predict, prevent, and respond to threats without human intervention. As AI continues to evolve, these systems will become more efficient, reducing the burden on cybersecurity professionals while strengthening digital defenses. These autonomous security frameworks will incorporate self-healing capabilities, automatically reconfiguring network architectures to eliminate vulnerabilities before they can be exploited.
By leveraging reinforcement learning. In conclusion,AI-powered analytics are shaping the future of cybersecurity by shifting from reactive to proactive threat detection. Innovations in anomaly detection, pattern recognition, and real-time threat intelligence are enhancing digital security.
As Dinesh Rajasekharan emphasizes, the integration of AI with emerging technologies will further strengthen cybersecurity defenses, ensuring a safer digital world..
Technology
AI-Powered Cybersecurity: The Future of Threat Detection
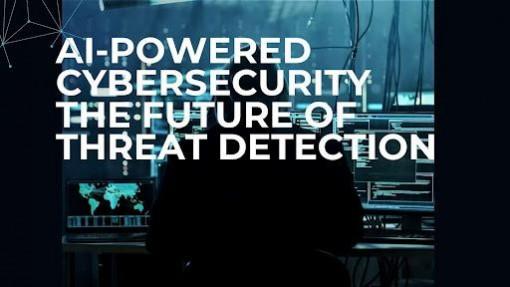
AI-powered analytics are shaping the future of cybersecurity by shifting from reactive to proactive threat detection