Imagine if policymakers could predict public responses to health interventions without costly and time-consuming human trials. This is no longer a distant possibility but an emerging reality thanks to advances in artificial intelligence (AI). A groundbreaking study, "Can A Society of Generative Agents Simulate Human Behavior and Inform Public Health Policy? A Case Study on Vaccine Hesitancy" explores the potential of generative agents to model vaccine hesitancy and shape public health strategies.
By creating a digital society of autonomous agents powered by Large Language Models (LLMs), researchers have attempted to understand and address one of the most persistent challenges in global healthcare. The study introduces VACSIM, an AI-driven simulation framework designed to model vaccine attitudes and predict the impact of public health interventions. The framework creates a virtual population of 100 generative agents, each assigned demographic attributes based on real-world census data.
These agents interact within a synthetic social network, forming relationships and influencing one another’s vaccine attitudes over time. The simulation exposes agents to vaccine-related news, social media content, and perceived disease risks, shaping their stance on vaccination through contextual interactions. The effectiveness of different vaccine-related policies - including mandates, financial incentives, and ambassador programs - is evaluated to determine how public attitudes shift in response.
To ensure realistic human-like behavior, the simulation incorporates attitude modulation, which adjusts agent perspectives dynamically to align with real-world hesitancy trends. This approach allows policymakers to explore potential interventions in a controlled environment before applying them in reality. Key findings: How AI simulations reveal trends in vaccine hesitancy Impact of public health policies on vaccine acceptance The study reveals that financial incentives, ambassador programs, and vaccine mandates each have different levels of effectiveness in reducing vaccine hesitancy.
Small financial incentives, such as a $10 reward, had minimal impact on changing attitudes, whereas larger incentives of $50 significantly increased vaccine acceptance, highlighting the importance of adequate motivation. Ambassador programs, where trained volunteers conducted personalized vaccine discussions, proved highly effective in convincing hesitant populations. However, efforts that lacked structured training had little influence on changing public perception.
Vaccine mandates were successful in increasing uptake but also faced strong resistance from certain demographic groups, demonstrating the challenge of balancing enforcement with public trust. Role of social networks in vaccine decision-making The findings emphasize the profound role of social networks in shaping vaccination attitudes. Agents who were surrounded by pro-vaccine peers gradually shifted towards acceptance, while those embedded in vaccine-hesitant communities reinforced each other’s skepticism.
This mirrors real-world patterns, where peer influence and community attitudes dictate public health behaviors. Additionally, exposure to misinformation further solidified vaccine hesitancy among certain groups, underscoring the necessity for effective health communication strategies that counteract false narratives and encourage evidence-based decision-making. How news and misinformation influence vaccine perception The type of news that generative AI agents consumed played a decisive role in shaping their vaccine attitudes.
Pro-vaccine news sources consistently reduced hesitancy, fostering trust in vaccination efforts. In contrast, exposure to vaccine-skeptical news amplified resistance, making individuals less likely to accept vaccines despite rising disease risks. These findings suggest that public health agencies must prioritize clear, fact-based messaging to combat misinformation and ensure that accurate information reaches hesitant populations before false narratives take hold.
Challenges and limitations of AI-driven vaccine hesitancy simulations Despite the promising potential of AI-generated simulations, several challenges remain. The study found that the simulation struggled to replicate certain real-world hesitancy trends, particularly among highly skeptical demographics. Some AI agents exhibited abrupt and unrealistic shifts in their vaccine attitudes, indicating that further refinements are needed to improve behavioral stability and accuracy.
Additionally, LLMs inherit biases from their training data, which may lead to skewed representations of human decision-making. These limitations underscore the need for continuous refinement of AI-driven simulations to ensure their reliability in informing real-world policy decisions. Future of AI in public health decision-making and vaccine outreach The implications of this study suggest that AI-powered generative agents could revolutionize public health policymaking.
By fine-tuning these simulations, governments can test different policy interventions before implementing them, reducing the risks associated with ineffective measures. Identifying the most vaccine-hesitant demographics allows for tailored outreach strategies that directly address specific concerns. Furthermore, improving vaccine communication strategies based on AI-driven insights can enhance public engagement and trust in vaccination programs.
The research marks a significant step toward integrating AI-driven insights into health policy, offering a glimpse into a future where digital simulations refine real-world interventions. While generative agents are not yet flawless, they present a powerful tool for optimizing vaccine policies, improving public health outreach, and ultimately saving lives through more effective, data-driven decision-making..
AI-driven vaccine hesitancy solutions: How generative agents shape public health policies
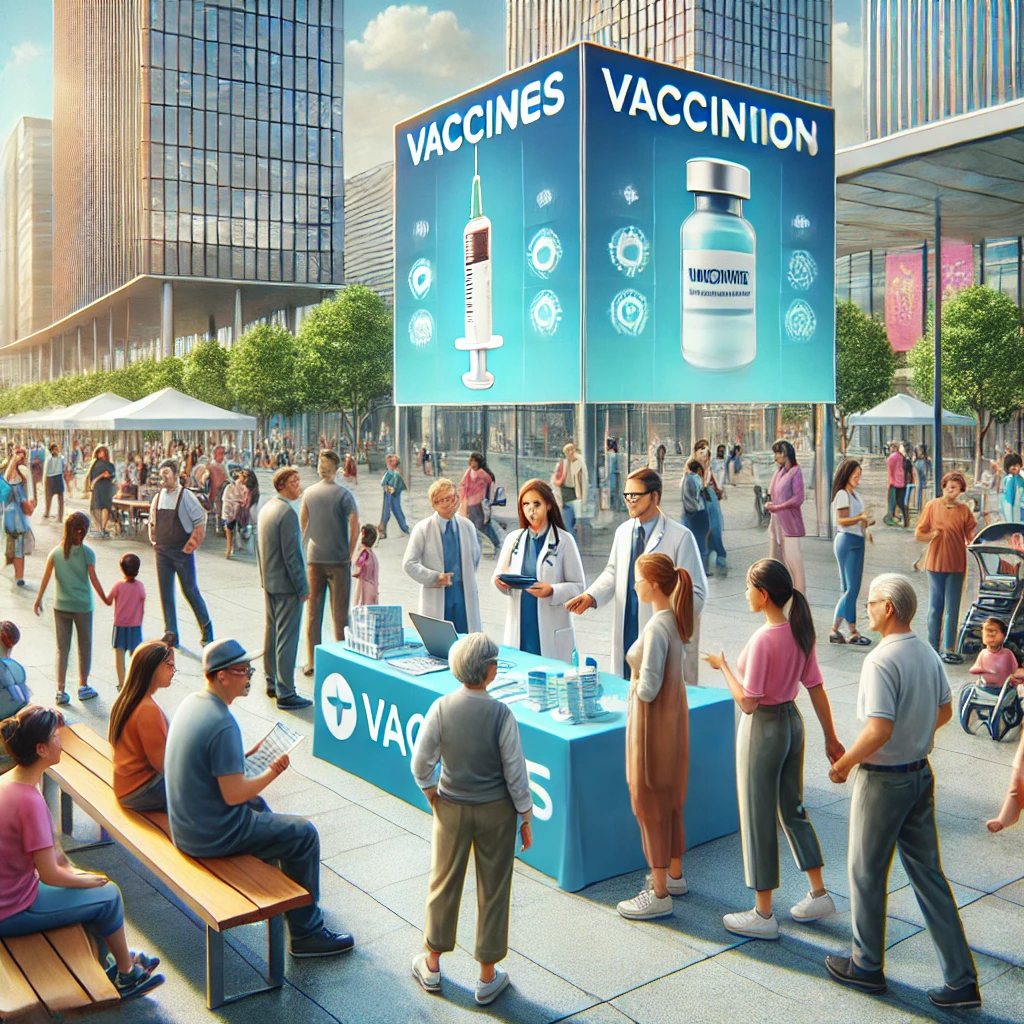
AI-driven vaccine hesitancy solutions: How generative agents shape public health policies Imagine if policymakers could predict public responses to health interventions without costly and time-consuming human trials. This is no longer a distant possibility but an emerging reality thanks to advances in artificial intelligence (AI). A groundbreaking study, "Can A Society of Generative Agents Simulate Human Behavior and Inform Public Health Policy? A Case Study on Vaccine Hesitancy" explores the potential of generative agents to model vaccine hesitancy and shape public health strategies. By creating a digital society of autonomous agents powered by Large Language Models (LLMs), researchers have attempted to understand and address one of the most persistent challenges in global healthcare.The study introduces VACSIM, an AI-driven simulation framework designed to model vaccine attitudes and predict the impact of public health interventions. The framework creates a virtual population of 100 generative agents, each assigned demographic attributes based on real-world census data. These agents interact within a synthetic social network, forming relationships and influencing one another's vaccine attitudes over time. The simulation exposes agents to vaccine-related news, social media content, and perceived disease risks, shaping their stance on vaccination through contextual interactions.The effectiveness of different vaccine-related policies - including mandates, financial incentives, and ambassador programs - is evaluated to determine how public attitudes shift in response. To ensure realistic human-like behavior, the simulation incorporates attitude modulation, which adjusts agent perspectives dynamically to align with real-world hesitancy trends. This approach allows policymakers to explore potential interventions in a controlled environment before applying them in reality.Key findings: How AI simulations reveal trends in vaccine hesitancyImpact of public health policies on vaccine acceptanceThe study reveals that financial incentives, ambassador programs, and vaccine mandates each have different levels of effectiveness in reducing vaccine hesitancy. Small financial incentives, such as a $10 reward, had minimal impact on changing attitudes, whereas larger incentives of $50 significantly increased vaccine acceptance, highlighting the importance of adequate motivation. Ambassador programs, where trained volunteers conducted personalized vaccine discussions, proved highly effective in convincing hesitant populations. However, efforts that lacked structured training had little influence on changing public perception. Vaccine mandates were successful in increasing uptake but also faced strong resistance from certain demographic groups, demonstrating the challenge of balancing enforcement with public trust.Role of social networks in vaccine decision-makingThe findings emphasize the profound role of social networks in shaping vaccination attitudes. Agents who were surrounded by pro-vaccine peers gradually shifted towards acceptance, while those embedded in vaccine-hesitant communities reinforced each other's skepticism. This mirrors real-world patterns, where peer influence and community attitudes dictate public health behaviors. Additionally, exposure to misinformation further solidified vaccine hesitancy among certain groups, underscoring the necessity for effective health communication strategies that counteract false narratives and encourage evidence-based decision-making.How news and misinformation influence vaccine perceptionThe type of news that generative AI agents consumed played a decisive role in shaping their vaccine attitudes. Pro-vaccine news sources consistently reduced hesitancy, fostering trust in vaccination efforts. In contrast, exposure to vaccine-skeptical news amplified resistance, making individuals less likely to accept vaccines despite rising disease risks.These findings suggest that public health agencies must prioritize clear, fact-based messaging to combat misinformation and ensure that accurate information reaches hesitant populations before false narratives take hold.Challenges and limitations of AI-driven vaccine hesitancy simulationsDespite the promising potential of AI-generated simulations, several challenges remain. The study found that the simulation struggled to replicate certain real-world hesitancy trends, particularly among highly skeptical demographics. Some AI agents exhibited abrupt and unrealistic shifts in their vaccine attitudes, indicating that further refinements are needed to improve behavioral stability and accuracy.Additionally, LLMs inherit biases from their training data, which may lead to skewed representations of human decision-making. These limitations underscore the need for continuous refinement of AI-driven simulations to ensure their reliability in informing real-world policy decisions.Future of AI in public health decision-making and vaccine outreachThe implications of this study suggest that AI-powered generative agents could revolutionize public health policymaking. By fine-tuning these simulations, governments can test different policy interventions before implementing them, reducing the risks associated with ineffective measures. Identifying the most vaccine-hesitant demographics allows for tailored outreach strategies that directly address specific concerns. Furthermore, improving vaccine communication strategies based on AI-driven insights can enhance public engagement and trust in vaccination programs.The research marks a significant step toward integrating AI-driven insights into health policy, offering a glimpse into a future where digital simulations refine real-world interventions. While generative agents are not yet flawless, they present a powerful tool for optimizing vaccine policies, improving public health outreach, and ultimately saving lives through more effective, data-driven decision-making.