Share Share Share Share Email In the modern digital era, cloud-native architectures form the backbone of enterprise IT, enabling scalability, flexibility, and seamless service delivery. However, as cloud environments grow in complexity, ensuring resilience, adaptability, and optimal performance becomes increasingly challenging. Artificial intelligence (AI) is emerging as a game-changer, transforming cloud-native systems by enhancing automation, predictive analytics, and real-time decision-making.
Prakash Ramesh , an expert in cloud computing and AI, has developed an innovative framework that integrates AI with performance and chaos engineering . This approach proactively addresses modern cloud challenges, ensuring robust, self-healing infrastructures that can withstand unpredictable failures and optimize efficiency. The Rise of Cloud-Native Systems Cloud-native architectures, leveraging microservices, containers, and dynamic orchestration, have transformed enterprise computing with enhanced scalability and flexibility.
However, they also introduce new challenges in performance and reliability. Traditional monitoring and testing methods are proving insufficient, driving the need for AI-driven automation to optimize system efficiency. As enterprises adopt these architectures, intelligent automation becomes crucial for managing complexity and ensuring seamless operations in modern cloud environments.
Automating Performance Engineering with AI Performance optimization in cloud environments requires real-time monitoring and rapid response to potential failures. AI-driven scheduling algorithms have significantly improved resource utilization by up to 83% and reduced task completion times by nearly 45%. Deep learning models, including LSTM networks and genetic algorithms, enhance cloud task scheduling by predicting workloads and adjusting resources dynamically, reducing operational costs and improving system reliability.
AI-Powered Real-Time Analytics Generative AI is reshaping real-time analytics by enabling faster decision-making and anomaly detection. AI-powered systems can process up to 850,000 events per second with a latency of under 100 milliseconds. Transformer-based models achieve 96.
7% accuracy in identifying performance anomalies, reducing false positive rates to as low as 2.3%. These capabilities allow organizations to detect potential system failures minutes before they occur, preventing disruptions and optimizing resource allocation.
Enhancing Chaos Engineering with AI Chaos engineering, a practice of intentionally introducing failures to test system resilience, is becoming smarter with AI integration. AI-driven fault detection mechanisms predict potential failures with up to 91.3% accuracy.
Real-time behavioral analysis enables machine learning models to respond instantly to anomalies, reducing mean time to recovery (MTTR) by 76% and improving service availability by 68%. Adaptive Fault Injection for System Resilience Traditional fault injection methods are static and inefficient. AI-powered adaptive fault injection dynamically adjusts testing patterns based on system responses, increasing test coverage by 73%.
Reinforcement learning algorithms identify critical components for testing, improving fault detection success rates by 85% while reducing the number of test scenarios required. This ensures that organizations maintain high system resilience without excessive manual intervention. Architecting AI-Driven Cloud Solutions Implementing AI in cloud-native architectures requires a multi-layered approach.
The data collection layer gathers system metrics with near-perfect reliability, while the processing layer transforms and normalizes data. The AI inference layer analyzes system behavior and provides insights in real time. Organizations adopting this approach have experienced a 73% reduction in system downtime and a 58% increase in resource allocation efficiency.
Scaling AI for Cloud-Native Environments Scalability remains a crucial challenge for cloud-based AI implementations. AI-driven optimization frameworks improve CPU utilization by 65% and memory management efficiency by 58%. Automated scaling mechanisms allow systems to handle loads that fluctuate by up to 300% while maintaining optimal response times.
This adaptability ensures consistent performance even under varying workloads. The Future of AI in Cloud Computing As cloud-native systems grow in complexity, AI’s role in performance and chaos engineering will continue to expand. By 2026, an estimated 95% of cloud-native applications will incorporate AI-driven optimization.
Future advancements in federated learning and AutoML will further enhance predictive capabilities, reducing deployment complexity and improving overall system efficiency. In conclusion, the integration of AI in performance and chaos engineering is transforming cloud-native architectures, enhancing their resilience, scalability, and efficiency. Organizations that adopt AI-driven automation witness significant improvements in system reliability and operational efficiency.
As AI technology continues to evolve, its role in optimizing cloud environments will become even more critical. Prakash Ramesh ’s work in this domain lays a strong foundation for the future of AI-powered cloud computing. Related Items: AI , Prakash Ramesh Share Share Share Share Email Recommended for you Revolutionizing Supply Chains: The Future of Inventory Tracking and AI Forecasting Revolutionizing Financial Reconciliation: The Power of AI and Human Collaboration Bridging AI Efficiency and Environmental Impact: A Path to Sustainable Innovation Comments.
Technology
AI-Driven Performance and Chaos Engineering: Reshaping Cloud-Native Architectures
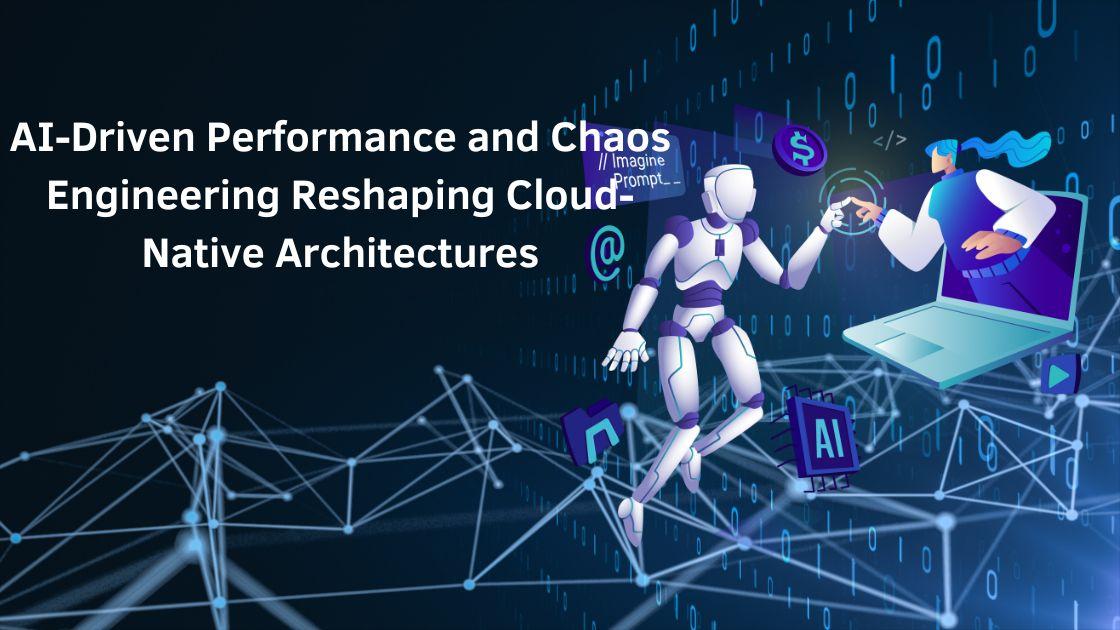
In the modern digital era, cloud-native architectures form the backbone of enterprise IT, enabling scalability, flexibility, and seamless service delivery. However, as cloud environments grow in complexity, ensuring resilience, adaptability, and optimal performance becomes increasingly challenging. Artificial intelligence (AI) is emerging as a game-changer, transforming cloud-native systems by enhancing automation, predictive analytics, and real-time decision-making. [...]The post AI-Driven Performance and Chaos Engineering: Reshaping Cloud-Native Architectures appeared first on TechBullion.